- 1ICAR-National Bureau of Plant Genetic Resources, New Delhi, India
- 2National Institute of Plant Genome Research, New Delhi, India
- 3ICAR- Indian Agricultural Statistics Research Institute, New Delhi, India
- 4ICAR-Vivekananda Parvatiya Krishi Anusandhan Sansthan, Almora, India
Ricebean (Vigna umbellata) is a lesser known pulse with well-recognized potential. Recently, it has emerged as a legume with endowed nutritional potential because of high concentration of quality protein and other vital nutrients in its seeds. However, the genes and pathways involved in regulating seed development and size are not understood in this crop. In our study, we analyzed the transcriptome of two genotypes with contrasting grain size (IC426787: large seeded and IC552985: small seeded) at two different time points, namely, 5 and 10 days post-anthesis (DPA). The bold seeded genotype across the time points (B5_B10) revealed 6,928 differentially expressed genes (DEGs), whereas the small seeded genotype across the time point (S5_S10) contributed to 14,544 DEGs. We have also identified several candidate genes for seed development–related traits like seed size and 100-seed weight. On the basis of similarity search and domain analysis, some candidate genes (PHO1, cytokinin dehydrogenase, A-type cytokinin, and ARR response negative regulator) related to 100-seed weight and seed size showed downregulation in the small seeded genotype. The MapMan and KEGG analysis confirmed that auxin and cytokinin pathways varied in both the contrasting genotypes and can therefore be the regulators of the seed size and other seed development–related traits in ricebeans. A total of 51 genes encoding SCFTIR1/AFB, Aux/IAA, ARFs, E3 ubiquitin transferase enzyme, and 26S proteasome showing distinct expression dynamics in bold and small genotypes were also identified. We have also validated randomly selected SSR markers in eight accessions of the Vigna species (V. umbellata: 6; Vigna radiata: 1; and Vigna mungo: 1). Cross-species transferability pattern of ricebean–derived SSR markers was higher in V. radiata (73.08%) than V. mungo (50%). To the best of our knowledge, this is the first transcriptomic study conducted in this crop to understand the molecular basis of any trait. It would provide us a comprehensive understanding of the complex transcriptome dynamics during the seed development and gene regulatory mechanism of the seed size determination in ricebeans.
Introduction
A rapid increase in the human population, which is expected to reach 9.7 billion by 2050, is one of the biggest challenges of this world (Gu et al., 2021). To ensure food and nutritional security to the ever-growing human population, it is extremely important to bring underutilized and neglected crops into mainstream agriculture. Owing to its short growth duration and ability to thrive well in stress conditions and various soil types, ricebean (Vigna umbellata) is one such crop which has huge potential to sustain food and nutritional security in most parts of the world (Pattanayak et al., 2019). It is a diploid (2n = 2× = 22), warm-season annual legume with a genome size of approximately 440 Mb (Kaul et al., 2019). Ricebean is mainly cultivated in Nepal, Bhutan, Northeast India up to Myanmar, Southern China, Northern Thailand, Laos, Vietnam, Indonesia, and East Timor (Tian et al., 2013), where it constitutes an important source of protein for the sizable population and contributes to household food and nutritional security. The observed protein content in ricebean is 25.57% with high concentration of various essential amino acids. Besides protein, ricebean grains also contain a significant amount of other nutrients such as carbohydrates, fiber, minerals, vitamins, and fatty acids. Moreover, ricebean is a rich source of unsaturated fatty acids like linoleic and linolenic acids (Katoch, 2013).
Among various productivity traits, pod length, seed size, and seed weight have major emphasis on ricebean genetic improvement programs because of their direct impact on the total grain yield. Furthermore, the seed is the key reservoir of proteins, essential amino acids, unsaturated fatty acids, and minerals in ricebean. Therefore, it is of great importance to decipher the molecular mechanism underlying seed development and size determination process in this minor but potential pulse crop. In recent years, with the advent of next-generation sequencing technology, key gene regulatory networks governing pod and seed development have been well characterized in both model plants like rice (Herridge et al., 2011), Arabidopsis (Herridge et al., 2011; Mahto et al., 2017), and also in non-model legume species like black gram (Souframanien and Reddy, 2015), cowpea (Lonardi et al., 2019), chickpea (Pradhan et al., 2014), mungbean (Tian et al., 2016a), and soybean (Jones and Vodkin, 2013; Qi et al., 2018; Peng et al., 2021). These studies revealed that seed development in higher plants is a highly complex process and governed by phytohormone signaling including cytokinins (CKs), gibberellins (GAs), brassinolides (BRs), ethylene (ET), and their associated genes and transcription factors. In all these phytohormones, genes related to auxin pathways including indole-3-acetic acid (IAA), auxin-responsive protein (IAA12, IAA), auxin response factors (ARFs), SAUR-like auxin superfamilies, auxin-related Aux/IAA, OsIAA18, and AP2/ERF, along with other genes such as ARR-B (cytokinin signaling), ethylene-responsive transcription factor (ERF084-like, ERF4, ERF061), ethylene-insensitive protein 3 (EIN3), ethylene receptor (ETR), ethylene-insensitive protein 4 (EIN4), serine/threonine-protein kinase (CTR1), ethylene responsive APATELA2 (AP2), ethylene-responsive element binding protein (EREBP), and many more genes were reported during seed development (Garg et al., 2011; Jones and Vodkin, 2013; Tian et al., 2016a; Nelson et al., 2017; Geng et al., 2018; Li et al., 2019a; Lonardi et al., 2019; Yi et al., 2019; Raizada and Jegadeesan, 2020; Zhu et al., 2020).
The aforementioned transcriptome-based gene expression analysis has provided a robust functional genomics resource for deciphering gene networks and candidate genes regulating various biological processes in crop plants. For minor crops with poorly characterized genomes, like ricebean, such detailed transcriptome analysis will provide comprehensive information about expression patterns of genes and molecular mechanisms governing traits of economic importance. This valuable information can further be employed for the development of functional markers for gene and QTL mapping. Therefore, in the present study, we conducted transcriptome analyses to investigate gene expression networks and identify the candidate genes controlling seed size variation in ricebean. RNA sequencing of two contrasting ricebean genotypes was performed at early development stages (i.e., 5 and 10 DPA). The study provides detailed insights into various gene networks and their potential roles in determining seed size. Furthermore, the study also identified simple sequence repeat (SSR) motifs that could be used for molecular mapping of seed size/weight and other related traits.
Materials and Methods
Plant Material and Growth Conditions
Seeds of two contrasting ricebean genotypes, namely, IC426787 (bold seeded) and IC552985 (small seeded) were obtained from ICAR-National Bureau of Plant Genetic Resources (NBPGR), New Delhi (Figure 1). On the basis of the 2-year trial (2018 and 2019), the average 100-seed weight of IC426787 and IC552985 was 13.20 and 3.87 gm, respectively. Plants were grown in a net-house at ICAR-NBPGR, New Delhi (latitude: 28°38′56″N, longitude: 77°9′8″E, altitude: 228 mean sea level (msl)), during Kharif (rainy) season 2020. During pod filling, the minimum temperature ranged from 10.8 to 23°C, maximum temperature ranged from 30.4 to 36°C, and average RH% varied from 53 to 56. The ricebean pod filling duration varied from 20 to 30 DPA depending upon the genotype. Genotypes with smaller grain size took comparatively less pod filling time than the genotypes having larger grain size. Three biological replicates of pod samples were harvested from three full-grown plants of both genotypes at 5 and 10 DPA each. The seeds were separated and immediately frozen in liquid nitrogen and stored at −80°C for future use. A total of 12 samples were prepared for the construction of RNA libraries.
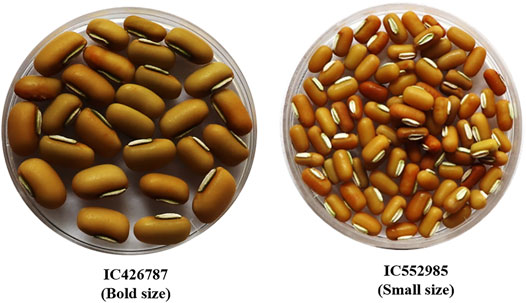
FIGURE 1. Two contrasting genotypes of ricebean, that is, IC426787 (bold size) and IC552985 (small size), selected for the transcriptome analysis on the basis of their seed size.
RNA Extraction, Library Preparation, and Sequencing
The Pure Link RNA Mini Kit (Ambion, United States) was used to extract RNA from the frozen samples. The total RNA quality was checked using the RNA 6000 Nano Kit (Agilent Technologies, United States) on a 2100 Bioanalyzer (Agilent Technologies, United States), with a minimum RNA integrity number (RIN) of 7. RNA concentrations were determined with a NanoDrop ND-8000 spectrophotometer (Nano-Drop Technologies, Thermo scientific, Wilmington, DE). RNA-Seq libraries for all samples were prepared using the NEBNext UltraII RNA library preparation kit for Illumina; Cat no: E7770 (New England Biolabs), according to manufacturers recommended protocol, and sequencing was done in a single HiSeq 4000 lane using 150 bp paired-end chemistry. Briefly, total RNA was used to purify poly (A) messenger RNA (mRNA) using oligo-dT labeled magnetic beads. Then, the isolated mRNA was fragmented into 200 to 500 bp pieces in the presence of divalent cations at 94°C for 5 min using an ultrasonicator. The cleaved RNA fragments were copied into first-strand cDNA using SuperScript-II reverse transcriptase (Life Technologies, Inc.) and random primers. After second-strand cDNA synthesis, fragments were end-repaired and A-tailed, and indexed adapters were ligated. The products were purified and enriched with PCR to create the final cDNA library. The tagged cDNA libraries were pooled in equal ratios and used for 2 × 150 bp paired-end sequencing on a single lane of the Illumina HiSeq 4000. Illumina clusters were generated and loaded onto the Illumina Flow Cell on the Illumina HiSeq 4000 instrument, and sequencing was carried out using 2 × 150 bp paired-end chemistry. After sequencing, the samples were demultiplexed, and the indexed adapter sequences were trimmed using CASAVA v1.8.2 software (Illumina Inc.).
Read Quality and Adapter Removal
Raw reads of ricebean were evaluated for their quality using FASTQC v0.11.8 package (http://www.bioinformatics.bbsrc.ac.uk/projects/fastqc/). Four parameters were considered: base quality score distribution, sequence quality score distribution, average base content per read, and GC distribution in the reads. Trimmomatic v0.36 was applied to remove the adapter and trim the low-quality reads (trimming includes reads with or without ambiguous sequence “N”) using default parameters. To correct the random sequencing errors in Illumina RNA-Seq reads, Rcorrector v1.0.3 was used. Clean reads were also checked for their quality using FASTQC only.
RNA-Seq De Novo Assembly and Transcriptome Assessment
The obtained clean reads of all 12 samples were assembled using Trinity v2.4.0 with the paired-end model and default K-mer value of 25. The de novo assembly was merged and clustered using CDHIT v4.0 to get non-redundant sequences. Furthermore, these non-redundant sequences were made transcripts using the trinity in-built script. The clean reads of each sample were mapped back to the de novo assembled genome through BWA-mem software with default parameters. The BAM files were handled by samtools. The number of reads mapped to genes was calculated using samtools v0.1.19. The expression difference of each transcript between different samples was calculated using DESeq2 R package. False discovery rate (FDR) values less than 0.01 and |log2 (fold change)| ≥2 were considered significant differences at the expression level. The transcript abundance was normalized by the fragments per kilobase of transcript per million mapped reads (FPKM) value.
Gene Functional Analysis
To annotate the assembled transcripts, sequences were aligned by BLASTX (e-value <1e−5) to protein databases, including the non-redundant protein (NR) database, Swiss-Prot, and Kyoto Encyclopedia of Genes and Genomes (KEGG) pathway database. A GO enrichment analysis was conducted for the transcripts according to biological process, cellular component, and molecular function ontologies using Blast2GO software (Liu et al., 2013; Calzadilla et al., 2016). The GO annotation functional classifications were determined using WEGO software for the distribution of gene functions (Ye et al., 2006). GO functional enrichment and KEGG pathway enrichment analysis were also tested at a significance cutoff of p-value. All the p-values were adjusted with the criterion of Bonferroni correction. We selected the corrected p-value of 0.05 as the threshold to determine significant enrichment terms of the gene sets. The MapMan analysis was also conducted to provide a graphical overview of the metabolic and regulatory pathways for the detected genes using the MapMan tool, and the mapping file of ricebean for all the samples was generated using the Mercator tool.
Candidate Gene Identification and Their Domain Analysis
The candidate genes for seed development-related traits were identified on the basis of similarity (BLASTX with similarity >80% and e-value <0.001) with genes responsible for similar traits in other species, including Arabidopsis, Phaseolus vulgaris, and Vigna species (V. radiata and V. angularis). Furthermore, the candidate genes were also validated in silico on the basis of their domain analysis. The amino acid sequences of the identified candidate genes were predicted and compared against the Pfam protein database using HMMER 3.0 (e-value ≤ 1e−10) to obtain candidate gene domain/family annotation information. A heatmap was also generated for the candidate genes on the basis of their expression in both the genotypes at different times of development. The heatmap was made using an in-house R script.
Simple Sequence Repeats Identification and Primer Design
The MIcroSAtellite (MISA) search engine (http://pgrc.ipk-gatersleben.de/misa) was employed for the identification of SSRs. The minimum numbers of repeats used for selecting the SSRs were ten for mononucleotide-based loci, six for dinucleotide loci, five for trinucleotide loci, and three for all larger repeat types (tetra- to hexanucleotide motifs). For validation, 50 SSR motifs were randomly selected, that is, 25 for dinucleotide and trinucleotide each. The primers for these selected SSR motifs were designed based on flanking sequences using Primer3 software (http://sourceforge.net/projects/primer3) with targeted size of PCR products in the range between 100 and 300 bp, primer length between 18 and 22 bp, GC content between 40 and 70, and melting temperature of 50–60°C.
Simple Sequence Repeats Validation
DNA was isolated from young leaves of eight accessions of Vigna species including V. umbellata (6), V. mungo (1), and V. radiata (1) by following the protocol described in the DNeasy plant mini kit (QIAGEN, Hilden, Germany). DNA concentration was measured using a NanoDrop™ 2000 spectrophotometer (Thermo, United States), and DNA quality was analyzed using 0.8% agarose gel. A working stock of DNA was (10 ng/µl) prepared with nuclease-free water for polymerase chain reaction (PCR) for SSR amplification.
For the SSR amplification, 20 µl reaction mixture containing 4 µl genomic DNA (40 ng), 10 µl Taq Polymerase 2X Master Mix (United States), 0.8 µl primers (10 pM), and 5.2 µl nuclease-free water were used. For the amplification, the following thermal conditions were carried out: initial denaturation of 94°C for 3 min, then 35 cycles of 94°C for 30 s, primer annealing at 55°C for 45 s, extension at 72°C for 1 min, and final extension for 10 min. PCR products were separated using high-resolution metaphor agarose gel (3%) electrophoresis. Furthermore, the dendrogram of the genotypes was generated using the hierarchical clustering algorithm in DARwin v6.0.21 software (https://darwin.cirad.fr/).
Results and Discussion
Transcriptome Sequencing and De Novo Assembly
To obtain a comprehensive transcriptome profile of ricebean 12 RNA libraries were sequenced, and a total of 94.35 Gb raw data were generated. For these 12 samples, approximately, 98.50–99.80% of reads passed the quality control, and 98.60–99.60% of the clean reads were mapped back to the de novo assembled ricebean genome. On average, raw data of the seed transcriptome at 5 DPA and 10 DPA had 50.33 and 48.66% GC content, respectively, while after trimming, the GC content of clean data at 5 DPA and 10 DPA was 48.66 and 49.33%, respectively, which is similar to the GC content reported in the previous study of ricebean (Chen et al., 2016; Table 1).
The obtained clean reads of all 12 samples were assembled using Trinity (v2.4.0) with default parameters. The assembled transcriptome consists of 218,486 super transcripts with an N50 value of 1,041. The number of transcripts generated in the current study is comparable to previous studies. In terms of N50, the ricebean had a higher N50 value than field pea (781) and chickpea (441) (Pradhan et al., 2014; Sudheesh et al., 2015) and less value than mungbean, common bean, and adzuki bean (Hiz et al., 2014; Chen et al., 2015b; Chen et al., 2016). These results indicate the good quality of ricebean transcriptome.
The lengths of the transcripts ranged from 201 to 15,828 bp, with an average length of 669 bp, which is less than other Vigna species like cowpea (871 bp) and mungbean (874 bp) but more than that of black gram (443 bp) (Chen et al., 2015b, 2017; Souframanien and Reddy, 2015). Of these transcripts, 146,622 (67.11%) were 201–500 bp; 39,620 (18.13%) were 501–1,000 bp; 12,654 (5.79%) were 1,001–1,500 bp; 6,511 (2.98%) were 1,501–2,000 bp; 3,986 (1.82%) were 2,001–2,500 bp; 2,567 (1.17%) were 2,501–3,000 bp; and 6,526 (2.99%) were more than 3,000 bp in length (Figure 2). The developed assembly showed ∼100% back mapping of total and important reads, and this shows that our assembly had vast and proper mapping quality for the generated reads. The high percentage of reads mapping back to the de novo assembled transcriptome is a quality metric that provides an assessment of assembly entirety (Hornett and Wheat, 2012).
Differential Expression Analysis
In this study, a comprehensive transcriptome analysis has been performed with the aim to reveal those gene expression changes that, independently of the genotype diversity, are involved in controlling seed size in ricebean. Comparative transcriptome analysis was performed between two genotypes with contrasting seed size at two time points, namely, 5 and 10 DPA. A similar type of study using two genotypes with a contrasting seed size has also been done in the peanut (Li et al., 2019b). The expression profile was checked for the individual genotypes across the time points (B5_B10, S5_S10) as well as between the genotypes at each time point (B5_S5, B10_S10) (Supplementary Table S1). False discovery rate (FDR) values less than 0.01 and |log2 (fold change) |≥2 were considered significant differences at the expression level.
While evaluating the expression difference individually for the bold genotype across the time point (B5_B10), 6,928 differentially expressed genes were identified. In B5_B10, the number of upregulated genes (6,284) were higher than downregulated genes (644), suggesting that these upregulated DEGs might be responsible for the increase in seed size. Similarly, a small genotype across the time point (S5_S10) contributed to 14,544 DEGs (Figure3A; Table 2). In contrast to B5_B10 expression results, S5_S10 had a high number of downregulated genes (7,862) in comparison with the upregulated genes (6,682), indicating that these downregulated genes might be repressing any transcriptional activity or downstream pathways resulting in the small size of ricebean seeds (Li et al., 2019b). To gain a better understanding of molecular processes/regulatory networks associated with the seed size in ricebeans, the pattern of differentially expressed genes was analyzed between genotypes in each time point and across the time point using a Venn diagram (Figure 3B).
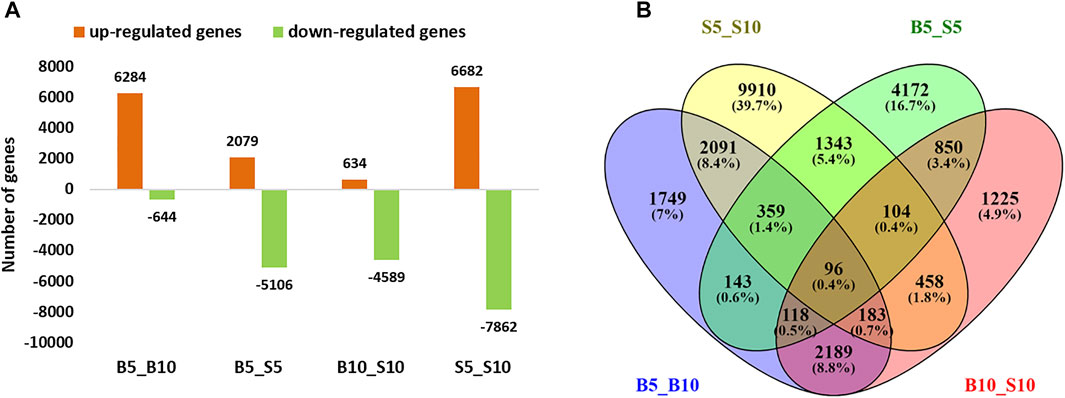
FIGURE 3. Differentially expressed genes in the two genotypes of ricebean at two time points, i.e., 5 and 10 DPA. (A) Comparison of DEGs representing the share of overlapped and non-overlapped transcripts in bold and small genotypes at 5 and 10 DPA. (B) Number of upregulated and downregulated significant genes in bold and small genotypes.
We also identified common genes between the individual genotype across time points (i.e., B5_B10 and S5_S10) as well as between the genotypes at each time point (B5_S5 and B10_S10). In case of B5_B10 and S5_S10, in total, 2091 DEGs were common. On the other hand, 850 DEGs were common between B5_S5 and B10_S10 (Supplementary Table S2). The comparative gene expression analysis indicated that a relatively large amount of the transcriptional program operating during seed development or maturation is shared between both the genotypes. The same results have been observed in the case of common bean, where 2,487 DEGs were shared by two contrasting genotypes (González et al., 2021).
Gene Ontology Analysis of the Transcriptome
To infer the biological processes and the functions related to seed development stages, gene ontology analysis was conducted for differentially expressed genes in terms of their biological involvement, target cellular component, and molecular function using Blast2GO. Out of total 33,880 DEGs, 16,002 DEGs contributed to GO terms. In core GO annotation, 7,002 (25.37%) genes annotated for biological process (BP), 12,069 (43.72%) for molecular function (MF), and 8,533 (30.91%) for cellular components (CC). The highest number of GO terms were observed in the case of S5_S10 (44.10%), followed by B5_B10 (23.89%), B10_S10 (16.91%), and B5_S5 (15.09%).
In case of the bold genotype across the time point (B5_B10), out of 6,928 DEGs, only 3,764 were annotated, constituting 1,696, 2,046, and 2,864 GO terms for BP, CC, and MF, respectively. However, in S5_S10, we observed 7,158 annotated DEGs from 14,544 DEGs and 2,954, 3,909, and 5,312 GO terms for BP, CC, and MF, respectively. On the other hand, in the case of between the genotype at the first time point (B5_S5), 2,537 DEGs were found to be annotated as compared B10_S10, in which 7,158 DEGs were annotated. In case of BP, 1,075 and 2,954 GO terms were identified in B5_S5 and S5_S10. Similarly, 2,046 and 3,909 GO terms were found for the cellular component function in B5_S5 and S5_S10, respectively, whereas in the case of molecular function, B5_S5 and S5_S10 consisted of 1,875 and 5,312 GO terms, respectively (Supplementary Table S3).
We have also illustrated the top or enriched functions in terms of BP, MF, and CC for both the genotypes. For example, the top biological activities include “cellular process,” “nitrogen compound metabolic process,” “small molecule metabolic process,” “cellular component organization,” “regulation of metabolic process,” “response to stress,” “cell wall organization,” cellular response to stimulus,” and developmental process. All these results indicated the biological process of DEGs vary over a broad range of terms. These enriched GO terms for BP indicate that hormone and environment stimuli played a vital role in ricebean seed/pod development. A similar type of results was also found in peanut pod development (Zhu et al., 2014).
Similarly, in the case of MF, bold and small genotypes were identified to be involved in “binding,” “metabolic processes,” “organic cyclic compound binding,” “heterocyclic compound binding,” “ion binding,” “transferase activity,” and “biosynthetic processes.” However, on the other hand, cellular component activities include “catalytic activity,” “membrane,” “membrane part,” “intrinsic component of the brain,” and “intracellular” and cellular activities” (Figure 4). Similar results for MF and CC were observed in the pod development of peanuts (Zhu et al., 2014).
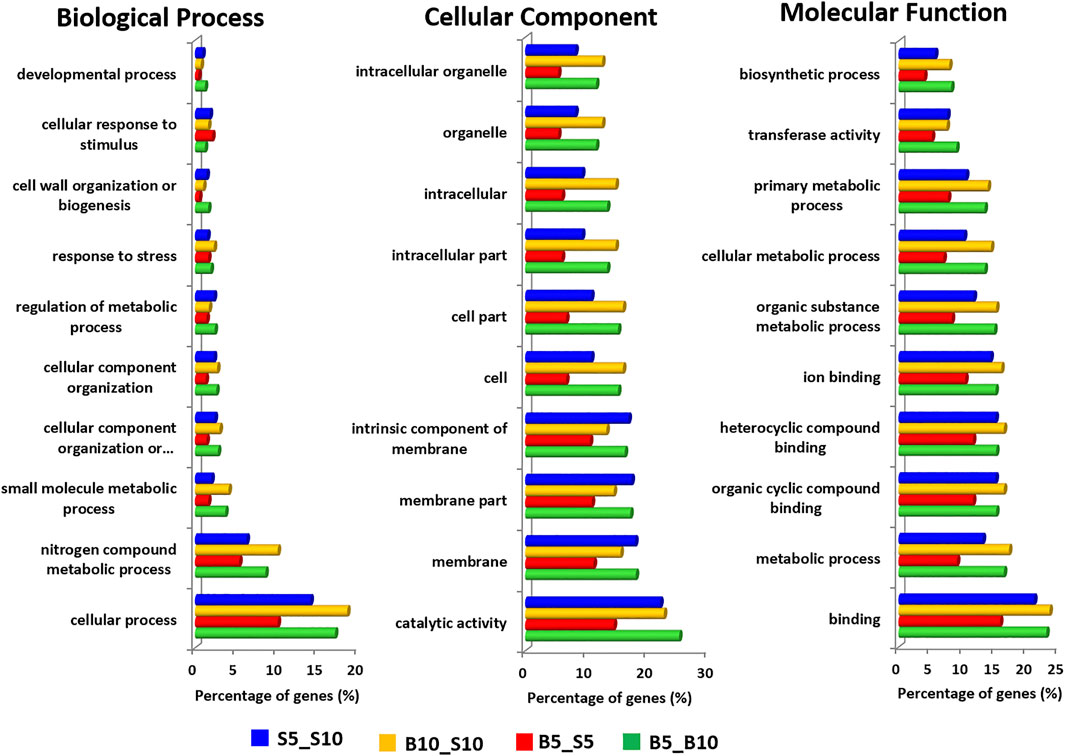
FIGURE 4. Gene ontology (GO) annotation of differentially expressed genes in ricebean summarized in three main categories: biological process, cellular component, and molecular function.
MapMan Analysis
For comprehensive assessment of gene expression network dynamics in a developing seed of bold and small genotypes, identified DEGs were mapped onto metabolic maps using the MapMan tool and categorized into BINS on the basis of their functional groups. We could observe various functional groups of genes activated at different stages of seed development. Interestingly, we noticed a major variation between the bold and small genotypes with respect to genes related to important functions like those involved in different aspects of metabolism and signaling or regulation. A detailed analysis of genes expressed in these categories that actually distinguish the two genotypes was considered relevant, and a major emphasis was therefore given to the BINS in which the genotypes were found to be involved. This analysis allowed exploration of the global activation of specific metabolic pathways and gene regulatory networks activated during ricebean seed development.
For the whole ricebean transcriptome, we annotated 13,759 transcripts with MapMan BINS of known function after running the Mercator web tool. In total, these transcripts were classified into 29 BINS. The transcripts were expressed mainly in the following categories: carbohydrate metabolism (major and minor CHO metabolism), amino acid turnover, photosynthesis, secondary metabolism, and cell wall organization (Supplementary Table S4). In the former categories, most of the transcripts were highly expressed in B5_B10, while downregulated in the case of S5_S10 (Figure 5A). B5_B10 and S5_S10 shared 17 pathways, but only two pathways were found in B5_B10 such as RNA processing and polyamine metabolism, indicating that these two pathways triggered after 5 DPA. Similarly, while comparing expressed transcripts between the genotype at the same time points (i.e., B5_S5 and B10_S10), 18 categories were the same, except the polyamine metabolism which was detected only at the second time point, that is, 10 DPA, which also confirms our previous result that polyamines activate only in the case of bold genotype after 5 DPA of seed development (Figure 6A).
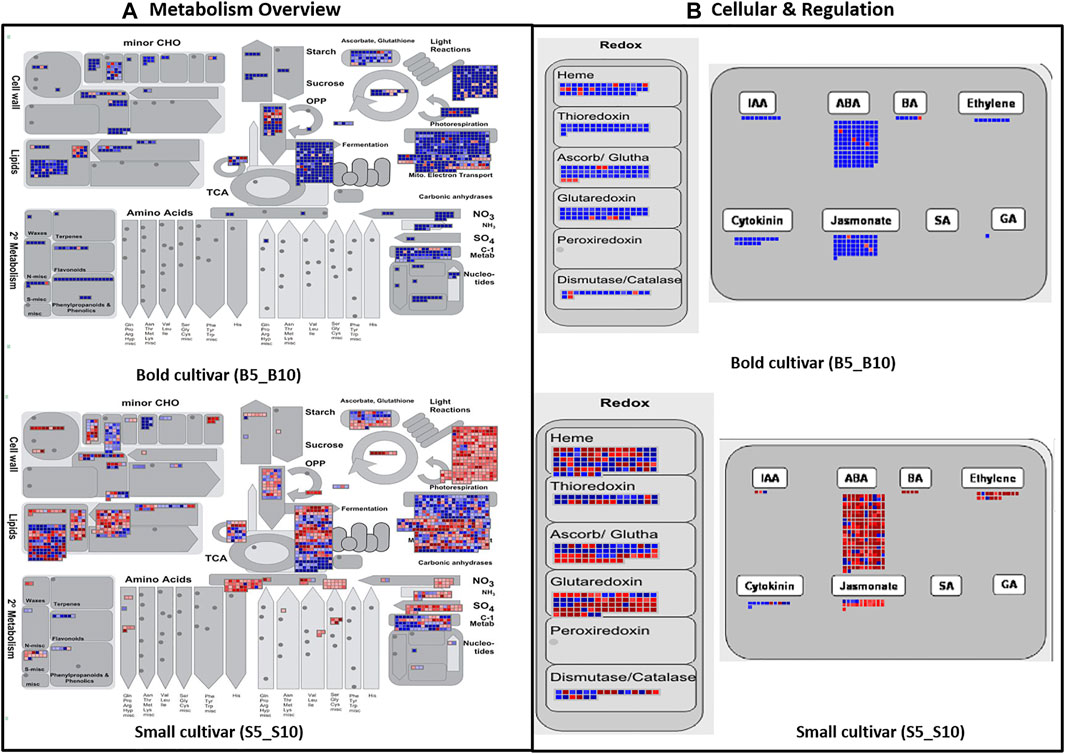
FIGURE 5. MapMan pathway representing the differential expression of genes across the time point involved in (A) metabolism (B) cellular and regulation pathway in bold and small genotypes.
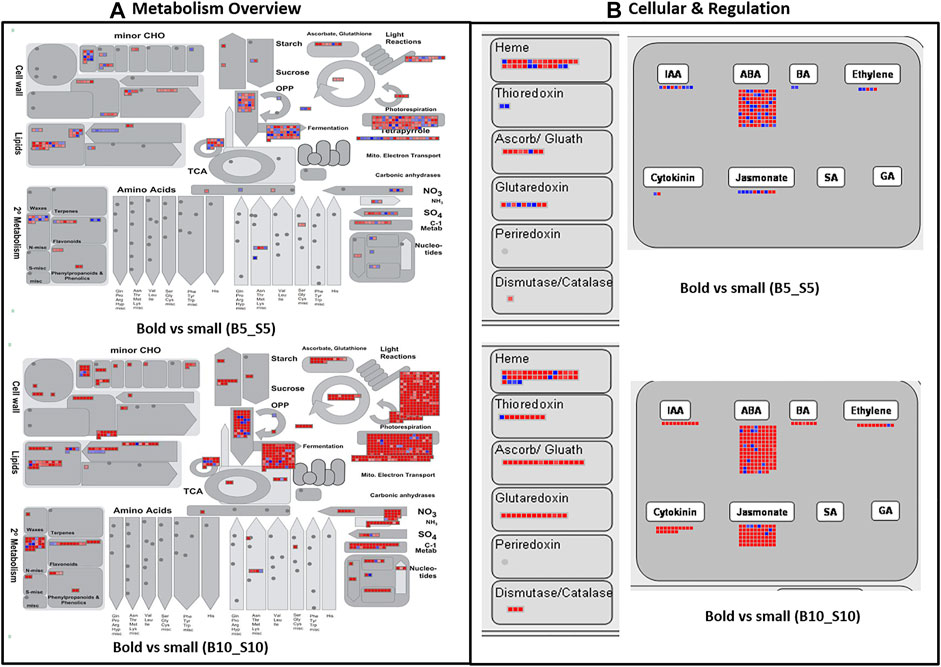
FIGURE 6. MapMan pathway representing the differential expression of genes across the genotype involved in (A) metabolism (B) cellular and regulation pathway in bold and small genotypes.
In our study, photosynthesis-related genes were highly enriched in bold genotypes in comparison with the small genotype which is in similarity with the previously published reports (Zhu et al., 2014; Clevenger et al., 2016; Sinha et al., 2020). The main role of photosynthesis in seed development is reported to increase the internal oxygen content and to control biosynthetic fluxes by improving the energy supply (Borisjuk et al., 2004), and it can also affect the metabolism in a number of distinct ways (Ruuska et al., 2004). Our results indicate that many metabolic genes are most active during ricebean seed filling, which aligns with previous studies on M. truncatula and P. sativa where approximately half of the seed-regulated genes were assigned to metabolic pathways (Benedito et al., 2008; Liu et al., 2015).
Furthermore, some DEGs are also mapped to hormone metabolic pathways. Majority of the genes associated with biosynthesis and response of many phytohormones like IAA, ABA, BAP, ethylene, cytokinin, jasmonate, and gibberellic acid were upregulated in the case of bold genotypes (B5_B10) as compared to small genotypes (S5_S10), in which most of the genes were downregulated (Supplementary Table S5; Figure 5B), whereas in case of B5_S5 and B10_S10, mixed expression of phytohormones was observed (Figure 6B); a complex regulatory network triggers the initiation of seed development, maturation, and accumulation of storage products. Several studies suggested the vital role of phytohormones in pod and seed development (Zhu et al., 2014; Huang et al., 2017; Wan et al., 2017; Kumar et al., 2019; Sinha et al., 2020). In 2017, a study demonstrated the role of phytohormones in various aspects of plant hormone homeostasis including biosynthesis, metabolism, receptor, and signal transduction (Xu and Huang, 2017).
Kyoto Encyclopedia of Genes and Genomes Pathway Analysis
The KEGG pathway enrichment analysis was conducted for two contrasting genotypes at both time points (i.e., 5 DPA and 10 DPA) at a p-value <0.05 using the KEGG database server. The KEGG pathway enrichment analysis indicated that 7,178 transcripts obtained hits in the KEGG database, and those transcripts were associated with 106 unique pathways. The 7,178 transcripts included 3,112, 434, 2,103, and 1,529 transcripts with respect to B5_B10, B5_S5, B10_S10, and S5_S10, respectively. The pathway enrichment analysis of DEG conducted between different combinations, B5_B10, B5_S5, B10_S10, and S5_S10, revealed involvement the of 7, 52, 458, and 35 pathways, respectively. In case of B5_B10 and S5_S10, from the top 10 pathways, four pathways, namely, biosynthesis of secondary metabolites, protein processing in the endoplasmic reticulum, plant–pathogen interaction, and starch and sucrose metabolism were common. On the other hand, between the genotypes at both the time points (i.e., B5_S5 and B10_S10), only one pathway i.e., metabolic pathway—was common. The top 10 pathways among the time points for both genotypes as well as between the genotypes at both the time points are represented in Figure 7.
In KEGG pathway–based analysis, we observed a clear difference in the expression of some phytohormones which regulates seed development, including auxin, cytokinin, gibberellin, and ethylene. The differential expression of these phytohormones was also observed in our MapMan analysis. This was not surprising since phytohormones control or influence all aspects of plant growth and reproduction, including seed germination, growth of roots, stems and leaves, plant flowering, seed development, seed fill, and seed dormancy. The expression pattern of key genes involved in biosynthesis and signaling of important phytohormones was compared between small and bold seeded genotypes for their possible role in determining seed size.
Auxin Pathway
Auxin regulates many aspects of plant growth and development, including embryogenesis (Möller and Weijers, 2009), the architecture of the root system (Benková et al., 2003), gravitropism (Rashotte et al., 2003), phototropism (Blakeslee et al., 2004), initiation and radial positioning of plant lateral organs, and cell elongation (Reinhardt et al., 2000; Christian et al., 2006). Auxin is sensed by its receptor protein such as TRANSPORT INHIBITOR RESPONSE 1/AUXIN-SIGNALING F-BOX proteins (TIR1/AFBs) which mediate the auxin signaling pathway and centered on a ubiquitin-dependent Skp1-Cullin-F-box (SCF)TIR1/AFBs protein complex to regulate the Aux/IAAs-ARFs flow (Leyser, 2003; Figure 8A). The TIR receptor protein confers substrate specificity and target-specific Aux/IAA proteins for degradation via the SCFTIR1/AFBs protein complex, in the presence of auxin. The degradation of Aux/IAA leads to switching on transcriptional expression of a range of genes including auxin responsive factors (ARFs) which in turn regulate the expression of several other genes that have a role in auxin-mediated plant growth and development.
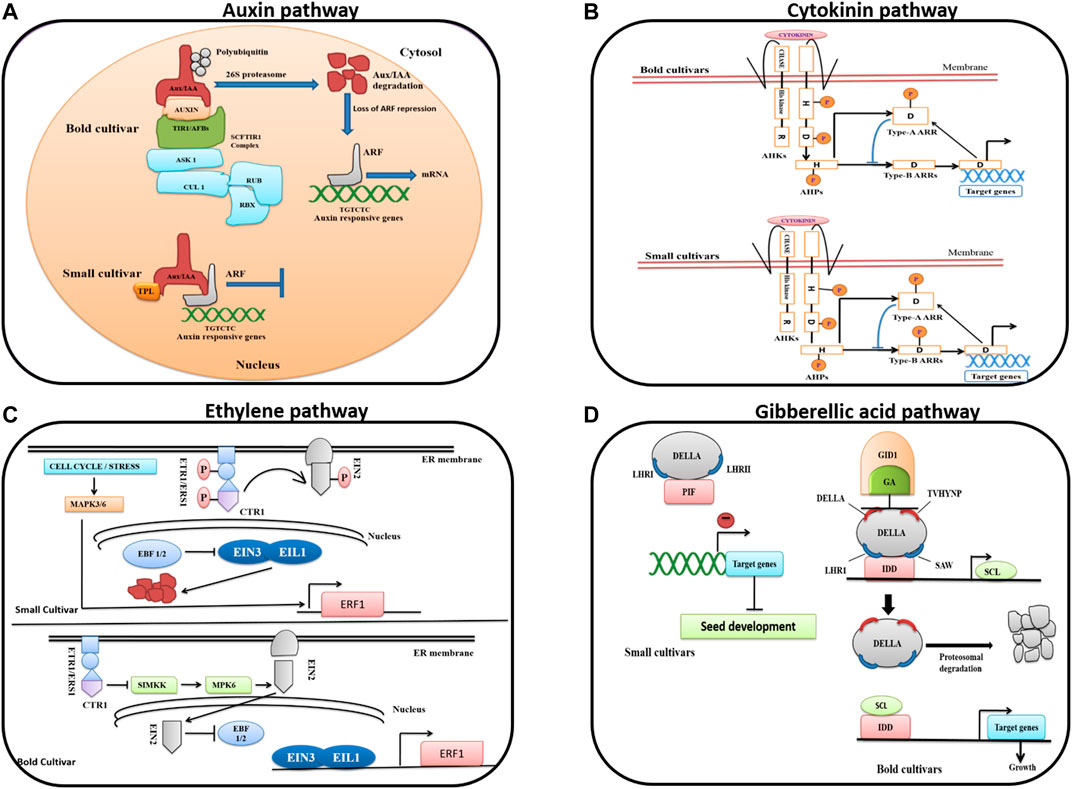
FIGURE 8. Phytohormone pathways important for seed development are represented in two contrasting genotypes of ricebeans on the basis of their expression and involvement in the enriched KEGG pathways. (A) Auxin signaling pathway. (B) Cytokinin pathway. (C) Ethylene pathway. (D) Gibberellic acid pathway.
The KEGG pathway expression–based analysis revealed a clear difference in the auxin signaling pathway in two contrasting ricebean genotypes, which is also in accordance with our MapMan results where auxin signaling related genes showed higher expression in the bold genotype than the small genotype. We found approximately 51 genes encoding SCFTIR1/AFB, Aux/IAA, ARFs, E3 ubiquitin transferase enzyme, and 26S proteasome, showing distinct expression dynamics in bold (B5_B10) and small (S5_S10) genotypes (Supplementary Table S6). The three key signaling elements TIR1/AFBs, Aux/IAAs, and ARFs have also been identified in different species including Arabidopsis (Chapman and Estelle, 2009), populus (Kalluri et al., 2007), and rice (Jain et al., 2005; Parry et al., 2009; Shen et al., 2010). Similarly, several studies focused on the role of AUX/IAA in determining the seed size with the influence of the expression of a gene in AUX biosynthesis (ZmTar3, ZmTar1, and ZmYuc1) and signaling (auxin efflux carriers, PIN, and ARF2) (Schruff et al., 2006; Bernardi et al., 2016). Homologs of ZmYuc1, PIN, and ARF2 were significantly differentially expressed during tartary buckwheat seed development (Huang et al., 2017). The high expression of DEGs in bold genotypes corresponds to cell division, and expansion is faster to form larger size seeds at these stages.
The upregulation of SCFTIR1, E3 ubiquitin transferase enzyme, and 26S proteasome was found in the bold genotypes, indicating the degradation of Aux/IAA and release of ARFs to modulate the expression of their target genes including SMALL AUXIN UP RNA (SAUR), Gretchen Hagen 3 (GH3), and indole-3-acetic acid–inducible gene (Aux/IAA), while in case of small genotypes, SCFTIR1 was not expressed, but TOPLESS (TPL) gene was upregulated, suggesting that Aux/IAA might have formed the complex with ARFs to block the transcriptional activity (Figure 8A; Hayashi, 2012).The induction of auxin-inducible acyl amidosynthetases, GH3, by the ARF family is the early event of auxin signaling cascade (Zhang et al., 2016). The expression of GH3 gene was upregulated in the case of bold genotypes, while it was downregulated in the small genotypes. SAUR expression was upregulated in small genotypes, while downregulated in bold genotypes. The aforementioned results clearly inferred that the differential regulation of the auxin signaling pathway in bold and small genotypes might be the main factor contributing to the variation in ricebean seed size (Bai et al., 2019).
Cytokinin Pathway
Similar to auxin, cytokinin is another important plant hormone regulating many aspects of plant growth (Tarkowski et al., 2006; Werner et al., 2008). In plants, the regulation of cytokinin is facilitated by the two-component system (TCS) which consists of four groups of proteins: histidine kinases (AHKs; AHK2, AHK3, and AHK4/WOL1/CRE1), histidine-containing phosphotransfer proteins (AHPs; AHP1-AHP5), type-B response regulators (type-B ARRs; ARR1, ARR2, ARR10-ARR14, and ARR18-ARR21), and type-A ARRs (ARR3-ARR9 and ARR15-ARR17). In Arabidopsis, AHK2, AHK3, and CRE1 were found to be involved in seed size (Riefler et al., 2006; Heyl et al., 2012).
Cytokinins have been reported to function in seed development, such as seed size, seed yield, embryonic growth, with the involvement of genes encoding isopentenyl transferase (IPT), cytokinin oxidase/dehydrogenase (CKX), and histidine kinase (HK) (Bartrina et al., 2011). In our study, we have also found the expression of genes such as IPT, CKX, and HK. IPT upregulation was observed only in the case of small genotypes, whereas CKX was upregulated in bold genotypes, and mixed expression of HK was noticed in both the genotypes (Figure 8B; Supplementary Table S7). The upregulation of CKX in bold seed genotypes hints at its possible role in determining the seed size. The CKX proteins are widely distributed in plants and implicated in various plant growth and developmental processes by maintaining the endogenous cytokinin level via irreversible degradation. In plant tissues, the expression of the CKX genes is primarily regulated by the endogenous cytokinin level. Various past studies have shown the role of CKX genes in the regulation of the seed size and grain yield in different plant species. In Arabidopsis, a CKX family gene–encoded enzyme CYTOKININ OXIDASE 2 (CKX 2) has been demonstrated to be associated with large seed size via catalyzing irreversible degradation of cytokinin. Similarly, in rice, a Gn1a locus encoding for cytokinin oxidase/dehydrogenase (OsCKX2) is shown to be responsible for high grain yield (Ashikari et al., 2005). On the other hand, the expression of type-A Arabidopsis response regulator (type-A ARRs) genes that negatively regulates the cytokinin signaling was majorly detected in small genotypes. This suggested that type-A ARR genes may be repressing the cytokinin signaling pathway (Heyl and Schmülling, 2003; Lohar et al., 2004; Desbrosses and Stougaard, 2011). The inhibition of the cytokinin signaling pathway may contribute to plant and bacterial cell differentiation (Bromley et al., 2014). Mixed expression of AHP and type-B ARRs was found in both the genotypes. Phosphate transfer to type-B ARR proteins modulates the transcriptional changes in the nucleus and causes the expression of primary cytokinin response genes including the type-B ARRs.
Ethylene Pathway
Ethylene, an “aging” hormone, has been reported to control the development of plant seeds and grains in various species (Zhong et al., 2002; Hentrich et al., 2013; Huang et al., 2013; Guo et al., 2016).Molecular evidence demonstrated ethylene’s role in the regulation of seed size and seed shape, in which genes in ethylene biosynthesis (EIN2, ERS1, and ETR1), signaling (CTR1, ETO1, ETR1, and EIN2), and catabolism (ACC deaminase) were involved (Robert et al., 2008; Walton et al., 2012). According to our results, the expression of ethylene receptors (ERS1/2) was higher in bold genotypes than small genotypes, whereas CTR1, a negative regulator of ethylene hormone showed contrasting expression with upregulation in small and downregulation in bold genotypes (Figure 8C; Supplementary Table S8). In buckwheat, the differential expression of ERS1, ETO1, ETR1, etc. was observed (Huang et al., 2017). In case of bold genotypes, we have noticed the high expression of SIMKK, MPK6, EIN3-like transcription factors, and EIN2, indicating positive regulation of transcriptional response in the bold genotype. In case of small genotypes, the upregulation of ERFs depicted that ERF might have shown activity after the phosphorylation via the MPK3/6-cascade, which regulates the ethylene biosynthesis, and the expression of EIN3/EIL1 was not found which possibly indicates its degradation by ubiquitination. In our samples, we found a full cascade of gene expressions in bold genotypes, while in small genotypes, the expression of genes detour from the normal expression and opted a new route for the ethylene-inducible gene expression.
Gibberellin Pathway
Gibberellins (GAs) are well-known plant hormones that are widely involved in the growth and development processes. GAs, auxin, ABA, and ethylene have been involved in the regulation of seed development and pod maturation (Ziv and Kahana, 1988; Shlamovitz et al., 1995; Ozga et al., 2003). In case of bold genotypes, the expression of GA, DELLAs, and SCF-complex protein is upregulated, which indicates DELLA proteolysis; simultaneously, the upregulation of protein indeterminate domains (IDDs) and scarecrow-like proteins (SCLs) were also observed, which supports the feedback loop mechanism which regulate the GA signaling (Figure 8D). According to the feedback loop mechanism, DELLA initiates the expression of downstream genes, including SCLs by IDD-mediated interaction with their promoters. The subsequent increased concentration of SCLs enhances the SCL3/IDD complex synthesis while decreasing the formation of the DELLA/IDD complex and consequent suppression of the expression of SCLs, which mediates the homeostatic regulation of the downstream genes, including positive regulation of SCLs and GA signaling. In case of small genotypes, the expression of SCF complex protein was absent, while the expression of DELLAs was unregulated. Consecutively, we observed the SCL protein script, while IDD protein was completely absent. The expression of the phytochrome-interacting factor (PIF) protein was found, which indicates the DELLA-mediated inhibition of hypocotyls elongation (Supplementary Table S9).
Previous studies have revealed that genes encoding GA2 oxidase and GA3 oxidase in the GA biosynthesis pathway can affect seed development, starch biosynthesis, embryo, and seed coat development (Nakayama et al., 2002; Singh et al., 2002). The downregulation of GA2-oxidase was observed in our results similar to a study of the tartary buckwheat in which the downregulation of GA2-oxidase was also depicted during seed development (Huang et al., 2017).
The KEGG pathway and the MapMan analysis suggested the differential expression of phytohormone biosynthesis or response genes. According to the MapMan analysis, auxin, cytokinin, ethylene, and gibberellin showed contrasting expressions in both the genotypes (Figure 5B). Similarly, in terms of the KEGG pathway, we have observed how the signaling pathways of these phytohormones were different. The present work confirms that auxin, cytokinin, ethylene, and gibberellin are the important regulators of the seed size in ricebean. Our results are also in accordance with those of previous studies in other species (Riefler et al., 2006; Heyl et al., 2012).
Candidate Gene Identification for Seed Development–Related Traits
The expression of a number of genes starting from the anthesis to early stages of maturity may have a crucial role in determining grain size and various other pod-related traits in pulses (Pazhamala et al., 2016). In this study, candidate genes for various traits such as days of flowering, pod shattering, seed per pod, seed size, 100-seed weight, and pod length were identified from the assembled transcriptome on the basis of sequence similarity search. In total, we identified 142 genes in ricebean belonging to development-related traits on the basis of similarity search (BLASTX) and e-value. Furthermore, the candidate genes were also characterized in silico on the basis of their domain analysis using Pfam software. Out of 142 genes, only 120 genes showed domain similarity with their hits. Therefore, we discarded 22 genes whose domain was not matched. Hence, according to our study, we found 120 candidate genes of ricebean belonging to different development-related traits (days of flowering, pod shattering, seed per pod, seed size, 100-seed weight, and seed length) (Figure 9; Supplementary Table S10).
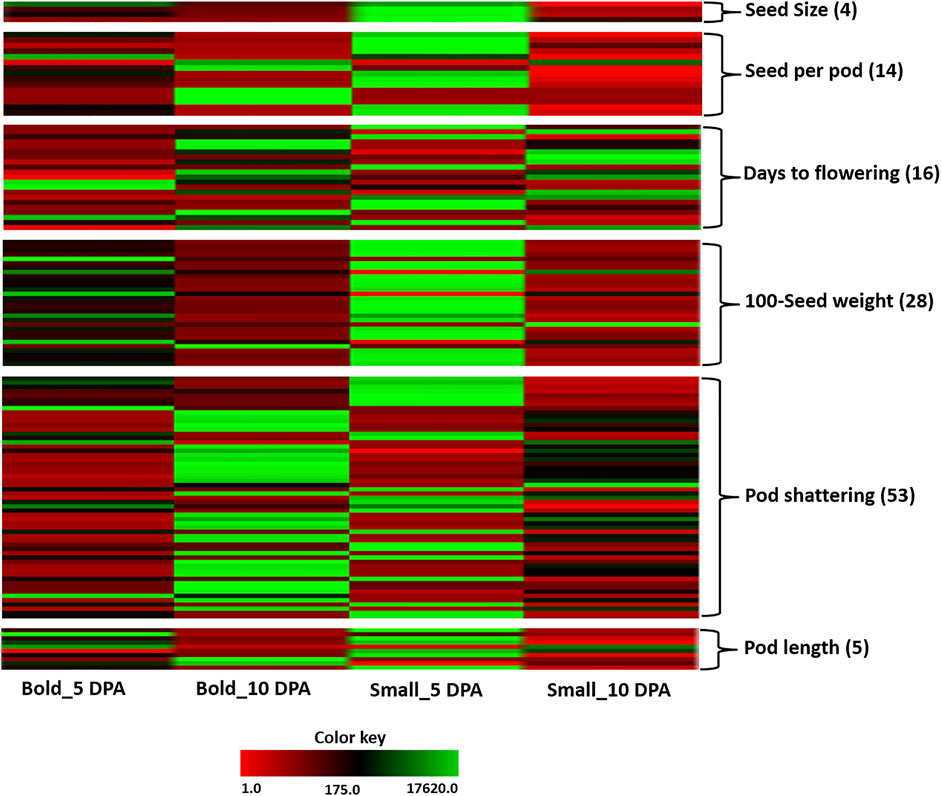
FIGURE 9. Heat map representing the differential gene expression of the identified candidate genes for six traits including seed size, 100-seed weight, seed/pod, days to flowering, pod shattering, and pod length in bold and small genotypes at 5 DPA and 10 DPA.
In terms of pod development, seed size is a key determinant for the seed or grain yield in legume crops (Amkul et al., 2020). In ricebean, we found four candidate genes for seed size encoding: histidine kinase 2, delta sterol reductase, phosphate transporter (PHO1), and WRKY domain–containing protein (WRKY 40). These genes have already been reported to be involved in seed size. For example, Vigun05g039600 (PHO1) has been reported to be a positive regulator of seed development that affects both the cell size and cell number (Lo et al., 2019). Similarly, Vigun08g217000 which codes for histidine kinase 2 has been identified as a potential candidate gene for improved organ size during cowpea domestication (Lonardi et al., 2019), and its Arabidopsis ortholog AHK2 has been shown to regulate the seed size (Riefler et al., 2006; Bartrina et al., 2017). Vigun11g191300 encoding a delta (24)-sterol reductase is an ortholog of the Arabidopsis DIMINUTO gene which has been shown to regulate cell elongation (Takahashi et al., 1995). In foxtail millet, Loose Panicle1–encoded WRKY transcription factor regulates the seed size by increasing the length and width of the seed (Xiang et al., 2017). Hence, these genes are the strong candidates as seed size is affected by multiple pathways.
On the other hand, for 100-seed weight, 29 candidate genes were identified corresponding to expansin, cytokinin dehydrogenase, cytochrome P450, and response regulatory domain containing protein. The significance of these genes as candidate loci related with the 100-seed weight is supported by the work done on Arabidopsis, where orthologs of the candidate genes in the cytokinin pathway have been shown, in transgenic studies, to regulate seed size and/or weight (Daele et al., 2012). Our findings are also in accordance with the common bean in which type-B regulators were found to be involved in the activation of downstream genes in the cytokinin pathway, and the genes encoding cytokinin dehydrogenase regulates the pathway by degrading active cytokinin (Hwang et al., 2012; Schmutz et al., 2014). Likewise, in Arabidopsis, expansins increased grain size and also improved grain production (Bae et al., 2014). Recent studies have also associated expansins with grain size and weight in wheat and tomato (Muñoz and Calderini, 2015; Brinton et al., 2017). TaCYP78A3 in wheat and CYP78A5 in Arabidopsis encodes the cytochrome P450, which positively correlates with seed size and seed weight (Ma et al., 2015; Tian et al., 2016b).
Like other development-related traits, flowering time is also an important trait because several agronomical traits such as quality of the grain and grain yield depend on flowering time. For days to flowering trait, we identified 21 candidate genes in our dataset encoding protein Flowering Locus T-like, GIGANTEA-like, cryptochrome, and transcription factors such as bHLH, ERF, and PIF-3. Most of the candidate genes of days to flowering had high expression in case of 10 DPA, instead of 5 DPA. In rice, florigen is encoded by RICE FLOWERING LOCUS T 1 (RFT1) and the orthologs of Arabidopsis FT and plays important role in heading date, influencing yield traits in rice (Tamaki et al., 2007; Komiya et al., 2009), whereas GIGNANTEA-like genes observed in the regulation of many genes which influence the circadian clock, blue light photoreceptor, and flowering time have also been reported in Arabidopsis (Hayama et al., 2003; Fornara et al., 2009). Similar to rice results, the Flowering Locust T-like in ricebean might help in the improvement of yield.
On the other hand, the number of seeds per pod might be useful for increasing the seed yield of ricebean. We identified 15 candidate genes for seeds per pod trait having annotations like MAPK, NAC, MALE STERILE 5, and ABSCISIC ACID-INSENSITIVE 5-like protein. Vigun03g187300 (ABA-insensitive 5-like protein 6) is an ABA-responsive element (ABRE)–binding factor that regulates ABRE-dependent gene expression (Nakashima and Yamaguchi-Shinozaki, 2013). In Arabidopsis, ABA deficiency reportedly decreases the number of seeds per siliqua (Cheng et al., 2014). Hence, the higher expression of this gene in bold genotypes implies an increase in the number of seeds per pod that could result in the improvement of the ricebean yield. The Vigun05g126900 gene, encoding MALE STERILE 5, was selected as a candidate gene in zombie pea (Amkul et al., 2020). In a previous study on Arabidopsis, mutations to MALE STERILE 5 resulted in the development of “polyads” (i.e., tetrads with more than four pools of chromosomes following male meiosis) (Glover et al., 1998). Plants that are homozygous for the MS5 recessive allele apparently revealed arrested growth and harvested empty siliques, whereas in plants that are heterozygous for MS5, siliqua elongation and seed set are less repressed (Glover et al., 1998). In case of the pod length, five candidate genes in ricebeans have been identified, mostly corresponding to the auxin response factor. Glyma.07G134800, an ortholog of Arabidopsis, was also associated with the auxin pathway (Jiang et al., 2018).
Furthermore, we have also identified a few candidate genes associated with pod shattering which is considered to be an undesirable agronomical trait. We identified maximum candidate genes (i.e., 57) for this trait in our ricebean study. Out of the 57 candidate genes, 18 genes encode transcription factors like AP2/ERF, WRKY, and NAC, whereas the rest of the genes were involved in cellulose synthase and serine/threonine protein kinase. The candidate genes for pod shattering have also been identified in other legumes including Vigun02g095200 (cellulose synthase), Vigun03g306000 (NAC domain transcription factor), and zombi pea (Suanum et al., 2016; Lo et al., 2018; Takahashi et al., 2019; Amkul et al., 2020; Watcharatpong et al., 2020). In Sorghum propinquum, WRKY modulates the flower and seed development and lignin deposition, and it is also found to be involved in pod shattering (Tang et al., 2013). Recently, in rice, AP2 transcription factor–coding gene SHATTERING ABORTION1 (SHAT1) was observed having a crucial role in pod shattering. Two genes encoding NAC in Vigna unguiculata were found to be involved in cell wall biosynthesis and hence influencing the pod shattering (Zhou et al., 2012; Lo et al., 2018). The identification of pod shattering genes may reduce preharvest yield damages in ricebean, resulting in a more efficient yield. Thus, pod indehiscence may be a valuable trait during seed harvesting, making it a main concern during crop domestication (Amkul et al., 2020).
To support our findings related to candidate genes, we performed a comparative analysis of the identified candidate genes with our MapMan and KEGG pathway results. Out of 120 candidate genes, 23 genes matched with the MapMan results (Table 3). For example, the expression of eight candidate genes of 100-seed weight and seed size were only shown in the small genotype encoding PHO1, cytokinin dehydrogenase, A-type cytokinin ARR response negative regulator, etc. Similarly, for bold genotypes, only one gene, aB10dtrinity_dn14585_c0_g1_i2, for a seed was upregulated, revealing a high number of pods in bold genotypes as compared to the small genotype. On the other hand, in terms of time point, three genes (cS5dtrinity_dn10996_c2_g4_i3: seed size; cS5dtrinity_dn11557_c0_g1_i4: seeds/pod; and aB10dtrinity_dn30303_c0_g10_i1: days to flowering) were detected only at the first time point, that is, 5 DPA. Two genes (aB10dtrinity_dn33078_c0_g1_i1: 100-seed weight and bS10d1trinity_dn10624_c1_g9_i1: pod shattering) were found to be highly expressed only in bold genotypes, whereas nine genes encoding alpha class expansins were found to be downregulated, specifically in the small genotype.
Similarly, 16 candidate genes (auxin: 2; cytokinin: 8; ethylene: 5; GA: 1) were matched with the KEGG pathway results (Table 4). The matched genes were found to be associated with several seed development–related traits like pod length, days to flowering, 100-seed weight, seeds/pod, and pod shattering. All the genes were expressed in the small genotype, except two (ab10dtrinity_dn29885_c1_g2_i2 and bs10dtrinity_dn12088_c3_g6_i6) which were expressed in bold genotypes corresponding to MAPK.
Simple Sequence Repeat Identification
In this study, we used the MISA Perl script (http://pgrc.ipk-gatersleben.de/misa) to detect the microsatellites. Of the 288,393 transcripts generated in this study, 14,663 contained an SSR totaling 201,517,181 bp. Out of these 14,663 sequences, 2,317 sequences had more than a single SSR, and 1,487 had SSRs of different motifs (compound SSR). Dinucleotide repeat motifs were the most abundant among the five types of motifs, totaling 8,866 (50.67%). The second most abundant were trinucleotides totaling 7,938 (45.36%), followed by 448 tetranucleotides (2.56%), 145 pentanucleotides (0.82%), and 100 hexanucleotide motifs (0.57%) (Figure 10A). Similar results have been reported in the previous transcriptome published for ricebean varieties (Chen et al., 2016) as well as for other legume species including mungbean (Tian et al., 2016b), adzuki bean (Chankaew et al., 2014; Chen et al., 2015b), cowpea (Gupta et al., 2010), and chickpea (Choudhary et al., 2008).
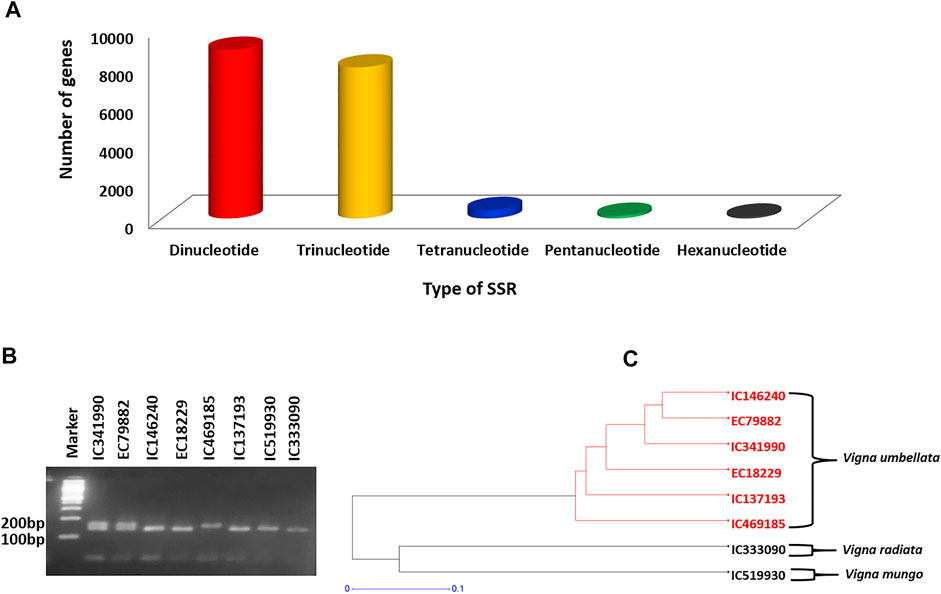
FIGURE 10. (A) Bar diagram representing the type and frequency of SSRs identified in ricebean using assembled transcripts. (B) SSR13 polymorphism on selected eight accessions of Vigna species. (C) Dendrogram representing the relationship distance among the eight accessions.
The number of the given repeat unit of SSRs ranged from 5 to >10, and as the number of repeat units increased, the frequency of the given SSR structure progressively decreased (Supplementary Table S11). As for the two most abundant repeat motif types (di- and trinucleotides), the frequency of the AG/CT motif type accounted for 17.41% in dinucleotide repeat motifs, and the frequency of GAA/TTC was the most abundant motif type in the trinucleotide, accounting for 6.3%. A previous study on adzuki bean also showed a high frequency of AG motifs in dinucleotides (Chankaew et al., 2014).
Simple Sequence Repeat Validation
To determine the polymorphism level of the identified EST-SSRs, the randomly selected 50 SSRs were evaluated in eight accessions of Vigna species including V. umbellata (6), V. mungo (1), and V. radiata (1) (Supplementary Table S12). From 50 pairs, 43 were successfully amplified, while seven pairs were not able to generate a PCR product (Supplementary Table S13). More than 85% of the SSR markers were successfully amplified, suggesting that the quality of our assembled transcripts was very high. The annealing temperatures of the primers ranged between 54 and 56°C. Out of these 43 SSR primer pairs, 26 pairs showed polymorphism (dinucleotide: 12, trinucleotide: 14) and the rest were monomorphic (Figure 10B; Table 5). A high polymorphism level (60.46%) of ricebean EST-SSRs was observed in the selected set of eight accessions which was higher than that from previous reports in other legume species including the chickpea (47.3%) (Nayak et al., 2010), mungbean (33%) (Chen et al., 2015b), black gram (58.2%) (Souframanien and Reddy, 2015), and adzuki bean (7.6%) (Chen et al., 2015a) while lower than common bean (71.3%) (Hanai et al., 2007), whereas when we considered only ricebean genotypes (six accessions), only 34.88% SSR markers were found polymorphic. We have also checked the cross-species transferability pattern and found that the transferability of ricebean–derived SSR markers was higher in V. radiata (73.08%) than in V. mungo (50%). Various studies depicted the importance of SSR cross-transferability in Vigna species including ricebean, mungbean, and cowpea (Pattanayak et al., 2019). Furthermore, the genetic distance among the accessions was determined, and we found two clusters, with six (V. umbellata) in the first cluster and two (V. mungo and V. radiata) in the second cluster, respectively (Figure 10C).
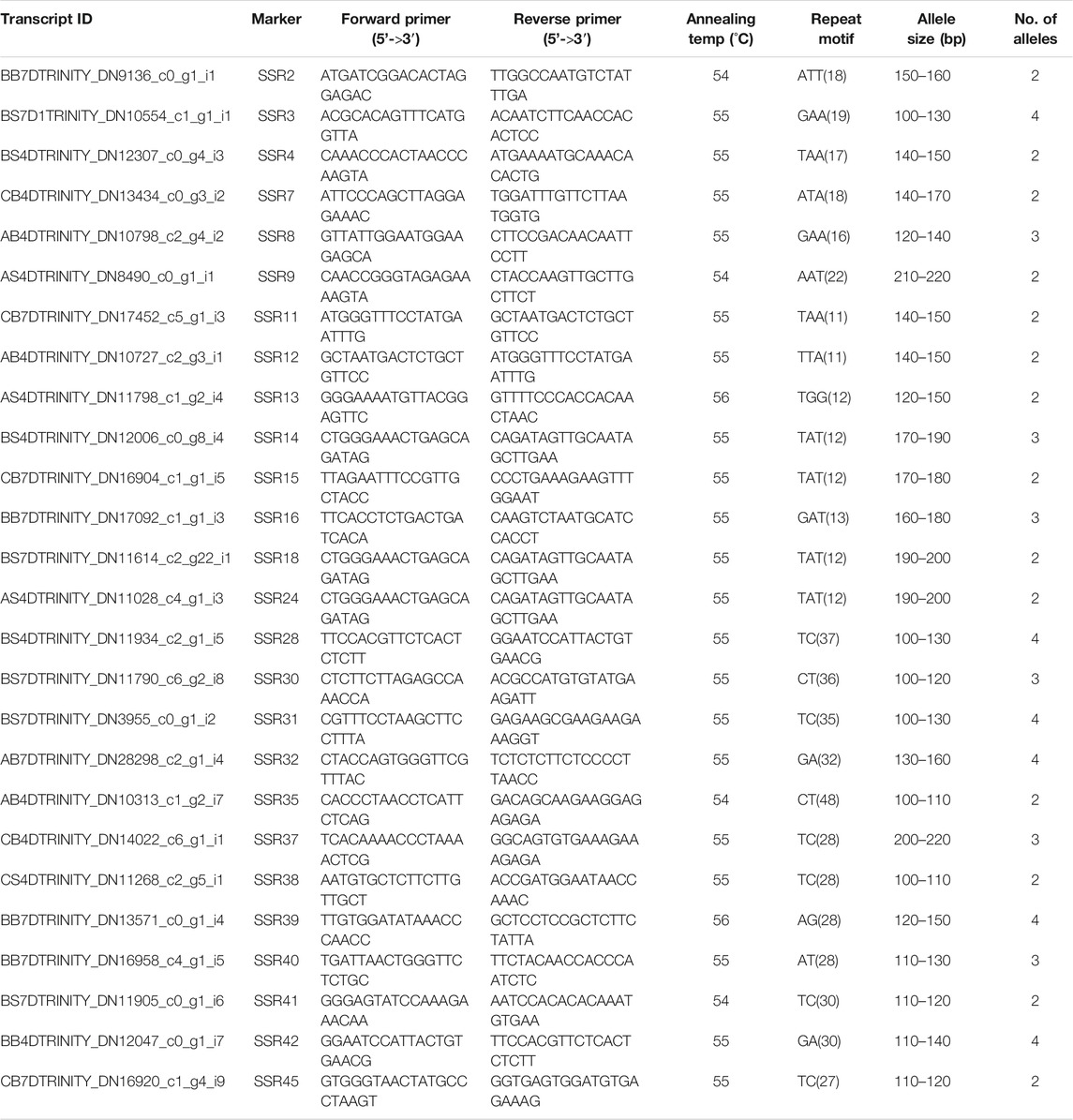
TABLE 5. List of 26 SSR markers that showed polymorphism in a set of eight accessions of Vigna species.
SSR molecular markers on the basis of transcriptomes have become more promising and useful because of their high cross-species transferability, their high rate of amplification, and being reasonably cheap as compared with the SSR markers of non-transcribed regions (Hansen et al., 2008; Rai et al., 2013). Moreover, since they can easily expose variance in the expressed portion of the genome, it is possible to evaluate marker–trait association (MTA) and specific genomic regions stating important physio-agronomic traits (Kalia et al., 2011).
Conclusion
The transcriptomic analysis in our study provided detailed insights into molecular processes and candidate genes controlling seed size and other seed development–related traits in ricebean. The MapMan and KEGG analyses confirmed that the phytohormone signaling pathways varied in both the contrasting expressions taken in this study and can therefore be the regulators of seed size as well as other seed development–related traits in ricebean. We hypothesize that the auxin, cytokinin, ethylene, and gibberellin signaling pathways interact cooperatively with one another, thereby modulating the expression of genes of seed development–related traits. Further research is required to identify key regulators/genes in determining seed size.
Data Availability Statement
The original contributions presented in the study are publicly available in NCBI under accession number PRJNA765494.
Author Contributions
SV contributed to investigation, formal analysis, and writing—original draft. SM helped with data curation, investigation, formal analysis, writing—original draft, and writing—review, and editing. GC contributed to resources and writing—review and editing. DW assisted with analysis and writing—review and editing. SP, DC, GP, DM, DJ, MS, and KS assisted with writing—review and editing. AS involved in conceptualization, supervision, resources, and writing—review and editing.
Funding
The financial support was received from the Department of Biotechnology under project: BT/Ag/Network/Pulses-1/2017-18.
Conflict of Interest
The authors declare that the research was conducted in the absence of any commercial or financial relationships that could be construed as a potential conflict of interest.
Publisher’s Note
All claims expressed in this article are solely those of the authors and do not necessarily represent those of their affiliated organizations, or those of the publisher, the editors, and the reviewers. Any product that may be evaluated in this article, or claim that may be made by its manufacturer, is not guaranteed or endorsed by the publisher.
Acknowledgments
The authors are also thankful to the Head of Division, Genomic Resources and Director, ICAR-NBPGR, New Delhi, for extending laboratory facilities for this work. The authors would also like to acknowledge the HPC facility at IACR-NBPGR for this work.
Supplementary Material
The Supplementary Material for this article can be found online at: https://www.frontiersin.org/articles/10.3389/fgene.2021.791355/full#supplementary-material
References
Amkul, K., Somta, P., Laosatit, K., and Wang, L. (2020). Identification of QTLs for Domestication-Related Traits in Zombi Pea [Vigna vexillata (L.) A. Rich], a Lost Crop of Africa. Front. Genet. 11, 803. doi:10.3389/FGENE.2020.00803
Ashikari, M., Sakakibara, H., Lin, S., Yamamoto, T., Takashi, T., Nishimura, A., et al. (2005). Cytokinin Oxidase Regulates Rice Grain Production. Science 309, 741–745. doi:10.1126/SCIENCE.1113373
Bae, J. M., Kwak, M. S., Noh, S. A., Oh, M. J., Kim, Y. S., and Shin, J. S. (2014). Overexpression of Sweetpotato Expansin cDNA (IbEXP1) Increases Seed Yield in Arabidopsis. Transgenic Res. 23, 657–667. doi:10.1007/S11248-014-9804-1
Bai, L., Chen, Q., Jiang, L., Lin, Y., Ye, Y., Liu, P., et al. (2019). Comparative Transcriptome Analysis Uncovers the Regulatory Functions of Long Noncoding RNAs in Fruit Development and Color Changes of Fragaria pentaphylla. Hortic. Res. 61 6, 1. doi:10.1038/s41438-019-0128-4
Bartrina, I., Jensen, H., Novák, O., Strnad, M., Werner, T., and Schmülling, T. (2017). Gain-of-Function Mutants of the Cytokinin Receptors AHK2 and AHK3 Regulate Plant Organ Size, Flowering Time and Plant Longevity. Plant Physiol. 173, 1783–1797. doi:10.1104/PP.16.01903
Bartrina, I., Otto, E., Strnad, M., Werner, T., and Schmülling, T. (2011). Cytokinin Regulates the Activity of Reproductive Meristems, Flower Organ Size, Ovule Formation, and Thus Seed Yield in Arabidopsis thaliana. Plant Cell 23, 69–80. doi:10.1105/TPC.110.079079
Benedito, V. A., Torres-Jerez, I., Murray, J. D., Andriankaja, A., Allen, S., Kakar, K., et al. (2008). A Gene Expression Atlas of the Model Legume Medicago truncatula. Plant J. 55, 504–513. doi:10.1111/J.1365-313X.2008.03519.X
Benková, E., Michniewicz, M., Sauer, M., Teichmann, T., Seifertová, D., Jürgens, G., et al. (2003). Local, Efflux-Dependent Auxin Gradients as a Common Module for Plant Organ Formation. Cell 115, 591–602. doi:10.1016/S0092-8674(03)00924-3
Bernardi, J., Li, Q.-B., Gao, Y., Zhao, Y., Battaglia, R., Marocco, A., et al. (2016). The Auxin-Deficient Defective Kernel18 (dek18) Mutation Alters the Expression of Seed-Specific Biosynthetic Genes in Maize. J. Plant Growth Regul. 353 (35), 770–777. doi:10.1007/S00344-016-9581-6
Blakeslee, J. J., Bandyopadhyay, A., Peer, W. A., Makam, S. N., and Murphy, A. S. (2004). Relocalization of the PIN1 Auxin Efflux Facilitator Plays a Role in Phototropic Responses. Plant Physiol. 134, 28–31. doi:10.1104/PP.103.031690
Borisjuk, L., Rolletschek, H., Radchuk, R., Weschke, W., Wobus, U., and Weber, H. (2004). Seed Development and Differentiation: A Role for Metabolic Regulation. Plant Biol. 6, 375–386. doi:10.1055/s-2004-817908
Brinton, J., Simmonds, J., Minter, F., Leverington-Waite, M., Snape, J., and Uauy, C. (2017). Increased Pericarp Cell Length Underlies a Major Quantitative Trait Locus for Grain Weight in Hexaploid Wheat. New Phytol. 215, 1026–1038. doi:10.1111/NPH.14624
Bromley, J. R., Warnes, B. J., Newell, C. A., Thomson, J. C. P., James, C. M., Turnbull, C. G. N., et al. (2014). A Purine Nucleoside Phosphorylase in Solanum tuberosum L. (Potato) with Specificity for Cytokinins Contributes to the Duration of Tuber Endodormancy. Biochem. J. 458, 225–237. doi:10.1042/BJ20130792
Calzadilla, P. I., Maiale, S. J., Ruiz, O. A., and Escaray, F. J. (2016). Transcriptome Response Mediated by Cold Stress in Lotus japonicus. Front. Plant Sci. 0, 374. doi:10.3389/FPLS.2016.00374
Chankaew, S., Isemura, T., Isobe, S., Kaga, A., Tomooka, N., Somta, P., et al. (2014). Detection of Genome Donor Species of Neglected Tetraploid Crop Vigna Reflexo-Pilosa (Créole Bean), and Genetic Structure of Diploid Species Based on Newly Developed EST-SSR Markers from Azuki Bean (Vigna angularis). PLoS One 9, e104990. doi:10.1371/JOURNAL.PONE.0104990
Chapman, E. J., and Estelle, M. (2009). Mechanism of Auxin-Regulated Gene Expression in Plants. Annu. Rev. Genet. 43, 265–285. doi:10.1146/annurev-genet-102108-134148
Chen, H., Chen, X., Tian, J., Yang, Y., Liu, Z., Hao, X., et al. (2016). Development of Gene-Based SSR Markers in Rice Bean (Vigna umbellata L.) Based on Transcriptome Data. PLoS One 11, e0151040. doi:10.1371/JOURNAL.PONE.0151040
Chen, H., Liu, L., Wang, L., Wang, S., Somta, P., and Cheng, X. (2015a). Development and Validation of EST-SSR Markers from the Transcriptome of Adzuki Bean (Vigna angularis). PLoS One 10, 1–14. doi:10.1371/journal.pone.0131939
Chen, H., Wang, L., Liu, X., Hu, L., Wang, S., and Cheng, X. (2017). De Novo transcriptomic Analysis of Cowpea (Vigna unguiculata L. Walp) for Genic SSR Marker Development. BMC Genet. 18, 1–12. doi:10.1186/S12863-017-0531-5
Chen, H., Wang, L., Wang, S., Liu, C., Blair, M. W., and Cheng, X. (2015b). Transcriptome Sequencing of Mung Bean (Vigna radiate L.) Genes and the Identification of EST-SSR Markers. PLoS One 10, e0120273. doi:10.1371/JOURNAL.PONE.0120273
Cheng, Z. J., Zhao, X. Y., Shao, X. X., Wang, F., Zhou, C., Liu, Y. G., et al. (2014). Abscisic Acid Regulates Early Seed Development in Arabidopsis by ABI5-Mediated Transcription of Short Hypocotyl Under Blue1. Plant Cell 26, 1053–1068. doi:10.1105/TPC.113.121566
Choudhary, S., Sethy, N. K., Shokeen, B., and Bhatia, S. (2008). Development of Chickpea EST-SSR Markers and Analysis of Allelic Variation across Related Species. Theor. Appl. Genet. 118, 591–608. doi:10.1007/S00122-008-0923-Z
Christian, M., Steffens, B., Schenck, D., Burmester, S., Böttger, M., and Lüthen, H. (2006). How Does Auxin Enhance Cell Elongation? Roles of Auxin-Binding Proteins and Potassium Channels in Growth Control. Plant Biol. 8, 346–352. doi:10.1055/S-2006-923965
Clevenger, J., Chu, Y., Scheffler, B., and Ozias-Akins, P. (2016). A Developmental Transcriptome Map for Allotetraploid Arachis hypogaea. Front. Plant Sci. 0, 1446. doi:10.3389/FPLS.2016.01446
Daele, I. V., Gonzalez, N., and Ilse, I. V. P. (2012). A Comparative Study of Seed Yield Parameters in Arabidopsis thaliana Mutants and Transgenics. Plant Biol. J. 10, 488–500. doi:10.1111/j.1467-7652.2012.00687.x
Desbrosses, G. J., and Stougaard, J. (2011). Root Nodulation: A Paradigm for How Plant-Microbe Symbiosis Influences Host Developmental Pathways. Cell Host Microbe 10, 348–358. doi:10.1016/J.CHOM.2011.09.005
Fornara, F., Panigrahi, K. C. S., Gissot, L., Sauerbrunn, N., Rühl, M., Jarillo, J. A., et al. (2009). Arabidopsis DOF Transcription Factors Act Redundantly to Reduce CONSTANS Expression and are Essential for a Photoperiodic Flowering Response. Dev. Cell 17, 75–86. doi:10.1016/J.DEVCEL.2009.06.015
Garg, R., Patel, R. K., Jhanwar, S., Priya, P., Bhattacharjee, A., Yadav, G., et al. (2011). Gene Discovery and Tissue-specific Transcriptome Analysis in Chickpea with Massively Parallel Pyrosequencing and Web Resource Development. Plant Physiol. 156, 1661–1678. doi:10.1104/pp.111.178616
Geng, X., Dong, N., Wang, Y., Li, G., Wang, L., Guo, X., et al. (2018). Correction: RNA-Seq Transcriptome Analysis of the Immature Seeds of Two Brassica Napus Lines with Extremely Different Thousand-Seed Weight to Identify the Candidate Genes Related to Seed Weight. PLoS ONE 13, 1. doi:10.1371/journal.pone.0218914
Glover, J., Grelon, M., Craig, S., Chaudhury, A., and Dennis, E. (1998). Cloning and Characterization of MS5 from Arabidopsis : A Gene Critical in Male Meiosis. Plant J. 15, 345–356. doi:10.1046/J.1365-313X.1998.00216.X
González, A. M., Yuste-Lisbona, F. J., Weller, J., Vander Schoor, J. K., Lozano, R., and Santalla, M. (2021). Characterization of QTL and Environmental Interactions Controlling Flowering Time in Andean Common Bean (Phaseolus vulgaris L.). Front. Plant Sci. 11, 599462. doi:10.3389/FPLS.2020.599462
Gu, D., Andreev, K., and Dupre, E. M. (2021). Major Trends in Population Growth Around the World. China CDC Wkly 3, 604–613. doi:10.46234/ccdcw2021.160
Guo, B., Wei, Y., Xu, R., Lin, S., Luan, H., Lv, C., et al. (2016). Genome-Wide Analysis of APETALA2/Ethylene-Responsive Factor (AP2/ERF) Gene Family in Barley (Hordeum vulgare L.). PLoS One 11, e0161322. doi:10.1371/JOURNAL.PONE.0161322
Gupta, P., Singh, R., Malhotra, S., Boora, K. S., and Singal, H. R. (2010). Characterization of Seed Storage Proteins in High Protein Genotypes of Cowpea [Vigna unguiculata (L.) Walp.]. Physiol. Mol. Biol. Plants 161, 53–58. doi:10.1007/S12298-010-0007-9
Hanai, L. R., De Campos, T., Camargo, L. E. A., Benchimol, L. L., De Souza, A. P., Melotto, M., et al. (2007). Development, Characterization, and Comparative Analysis of Polymorphism at Common Bean SSR Loci Isolated from Genic and Genomic Sources. Genome 50, 266–277. doi:10.1139/G07-007
Hansen, B. G., Halkier, B. A., and Kliebenstein, D. J. (2008). Identifying the Molecular Basis of QTLs: eQTLs Add a New Dimension. Trends Plant Sci. 13, 72–77. doi:10.1016/J.TPLANTS.2007.11.008
Hayama, R., Yokoi, S., Tamaki, S., Yano, M., and Shimamoto, K. (2003). Adaptation of Photoperiodic Control Pathways Produces Short-Day Flowering in Rice. Nature 422, 719–722. doi:10.1038/nature01549
Hayashi, K. (2012). The Interaction and Integration of Auxin Signaling Components. Plant Cell Physiol. 53, 965–975. doi:10.1093/PCP/PCS035
Hentrich, M., Sánchez-Parra, B., Alonso, M.-M. P., Loba, V. C., Carrillo, L., Vicente-Carbajosa, J., et al. (2013). YUCCA8 and YUCCA9 Overexpression Reveals a Link between Auxin Signaling and Lignification through the Induction of Ethylene Biosynthesis, Plant Signal. Behav. 8, e26363. doi:10.4161/psb.26363
Herridge, R. P., Day, R. C., Baldwin, S., and Macknight, R. C. (2011). Rapid Analysis of Seed Size in Arabidopsis for Mutant and QTL Discovery. Plant Methods 71 (7), 1. doi:10.1186/1746-4811-7-3
Heyl, A., Riefler, M., Romanov, G. A., and Schmülling, T. (2012). Properties, Functions and Evolution of Cytokinin Receptors. Eur. J. Cell Biol. 91, 246–256. doi:10.1016/J.EJCB.2011.02.009
Heyl, A., and Schmülling, T. (2003). Cytokinin Signal Perception and Transduction. Curr. Opin. Plant Biol. 6, 480–488. doi:10.1016/S1369-5266(03)00087-6
Hiz, M. C., Canher, B., Niron, H., and Turet, M. (2014). Transcriptome Analysis of Salt Tolerant Common Bean (Phaseolus vulgaris L.) under Saline Conditions. PLoS One 9, e92598. doi:10.1371/JOURNAL.PONE.0092598
Hornett, E. A., and Wheat, C. W. (2012). Quantitative RNA-Seq Analysis in Non-Model Species: Assessing Transcriptome Assemblies as a Scaffold and the Utility of Evolutionary Divergent Genomic Reference Species. BMC Genom. 13, 361. doi:10.1186/1471-2164-13-361
Huang, J., Deng, J., Shi, T., Chen, Q., Liang, C., Meng, Z., et al. (2017). Global Transcriptome Analysis and Identification of Genes Involved in Nutrients Accumulation during Seed Development of rice Tartary Buckwheat (Fagopyrum tararicum). Sci. Rep. 71, 1–14. doi:10.1038/s41598-017-11929-z
Huang, R., Jiang, L., Zheng, J., Wang, T., Wang, H., Huang, Y., et al. (2013). Genetic Bases of rice Grain Shape: So many Genes, So Little Known. Trends Plant Sci. 18, 218–226. doi:10.1016/J.TPLANTS.2012.11.001
Hwang, I., Sheen, J., and Müller, B. (2012). Cytokinin Signaling Networks. Annu. Rev. Plant Biol. 63, 353. doi:10.1146/annurev-arplant-042811-105503
Jain, M., Kaur, N., Garg, R., Thakur, J. K., Tyagi, A. K., and Khurana, J. P. (20052005). Structure and Expression Analysis of Early Auxin-Responsive Aux/IAA Gene Family in Rice (Oryza sativa). Funct. Integr. Genom. 61 6, 47–59. doi:10.1007/S10142-005-0005-0
Jiang, H., Li, Y., Qin, H., Li, Y., Qi, H., Li, C., et al. (2018). Identification of Major QTLs Associated with First Pod Height and Candidate Gene Mining in Soybean. Front. Plant Sci. 0, 1280. doi:10.3389/FPLS.2018.01280
Jones, S. I., and Vodkin, L. O. (2013). Using RNA-Seq to Profile Soybean Seed Development from Fertilization to Maturity. PLoS One 8. doi:10.1371/journal.pone.0059270
Kalia, R. K., Rai, M. K., Kalia, S., Singh, R., and Dhawan, A. K. (2011). Microsatellite Markers: An Overview of the Recent Progress in Plants. Euphytica 177, 309–334. doi:10.1007/s10681-010-0286-9
Kalluri, U. C., DiFazio, S. P., Brunner, A. M., and Tuskan, G. A. (2007). Genome-Wide Analysis of Aux/IAA and ARF Gene Families in Populus trichocarpa. BMC Plant Biol. 71 (7), 1. doi:10.1186/1471-2229-7-59
Katoch, R. (2013). Nutritional Potential of Rice Bean (Vigna umbellata): An Underutilized Legume. J. Food Sci. 78, 8–16. doi:10.1111/j.1750-3841.2012.02989.x
Kaul, T., Eswaran, M., Thangaraj, A., Meyyazhagan, A., Nehra, M., Raman, N. M., et al. (2019). Rice Bean (Vigna umbellata) Draft Genome Sequence: Unravelling the Late Flowering and Unpalatability Related Genomic Resources for Efficient Domestication of This Underutilized Crop. bioRxiv, 816595. doi:10.1101/816595
Komiya, R., Yokoi, S., and Shimamoto, K. (2009). A Gene Network for Long-Day Flowering Activates RFT1 Encoding a mobile Flowering Signal in Rice. Development 136, 3443–3450. doi:10.1242/DEV.040170
Kumar, A., Patel, J. S., Meena, V. S., and Srivastava, R. (2019). Recent Advances of PGPR Based Approaches for Stress Tolerance in Plants for Sustainable Agriculture. Biocatal. Agric. Biotechnol. 20, 101271. doi:10.1016/J.BCAB.2019.101271
Leyser, O. (2003). Molecular Genetics of Auxin Signaling. Annu. Rev. Plant Biol. 53, 377. doi:10.1146/annurev.arplant.53.100301.135227
Li, H., Lv, Q., Deng, J., Huang, J., Cai, F., Liang, C., et al. (2019a). Transcriptome Analysis Reveals Key Seed-Development Genes in Common Buckwheat (Fagopyrum esculentum). Int. J. Mol. Sci. 20, 4303. doi:10.3390/ijms20174303
Li, N., Wang, Y., Lu, J., and Liu, C. (2019b). Genome-Wide Identification and Characterization of the ALOG Domain Genes in Rice. Int. J. Genomics 2019, 2146391. doi:10.1155/2019/2146391
Liu, N., Zhang, G., Xu, S., Mao, W., Hu, Q., and Gong, Y. (2015). Comparative Transcriptomic Analyses of Vegetable and Grain Pea (Pisum sativum L.) Seed Development. Front. Plant Sci. 0, 1039. doi:10.3389/FPLS.2015.01039
Liu, T., Zhu, S., Tang, Q., Chen, P., Yu, Y., and Tang, S. (2013). De Novo Assembly and Characterization of Transcriptome Using Illumina Paired-End Sequencing and Identification of CesA Gene in Ramie (Boehmeria nivea L. Gaud ). BMC Genomics 14, 125. doi:10.1186/1471-2164-14-125
Lo, S., Muñoz-Amatriaín, M., Boukar, O., Herniter, I., Cisse, N., Guo, Y.-N., et al. (2018). Identification of QTL Controlling Domestication-Related Traits in Cowpea (Vigna unguiculata L. Walp). Sci. Rep. 81 8, 1–9. doi:10.1038/s41598-018-24349-4
Lo, S., Muñoz-Amatriaín, M., Hokin, S. A., Cisse, N., Roberts, P. A., Farmer, A. D., et al. (2019). A Genome-wide Association and Meta-Analysis Reveal Regions Associated with Seed Size in Cowpea [Vigna unguiculata (L.) Walp]. Theor. Appl. Genet. 132, 3079–3087. doi:10.1007/S00122-019-03407-Z
Lohar, D. P., Schaff, J. E., Laskey, J. G., Kieber, J. J., Bilyeu, K. D., and Bird, D. M. (2004). Cytokinins Play Opposite Roles in Lateral Root Formation, and Nematode and Rhizobial Symbioses. Plant J. 38, 203–214. doi:10.1111/J.1365-313X.2004.02038.X
Lonardi, S., Muñoz-Amatriaín, M., Liang, Q., Shu, S., Wanamaker, S. I., Lo, S., et al. (2019). The Genome of Cowpea (Vigna unguiculata [L.] Walp.). Plant J. 98, 767–782. doi:10.1111/TPJ.14349
Ma, M., Wang, Q., Li, Z., Cheng, H., Li, Z., Liu, X., et al. (2015). Expression of TaCYP78A3, a Gene Encoding Cytochrome P450 CYP78A3 Protein in Wheat (Triticum aestivum L.), Affects Seed Size. Plant J. 83, 312–325. doi:10.1111/TPJ.12896
Mahto, A., Mathew, I. E., and Agarwal, P. (2017). Decoding the Transcriptome of Rice Seed During Development. Adv. Seed Biol., 25 doi:10.5772/INTECHOPEN.70574
Möller, B., and Weijers, D. (2009). Auxin Control of Embryo Patterning. Cold Spring Harb. Perspect. Biol. 1, a001545. doi:10.1101/CSHPERSPECT.A001545
Muñoz, M., and Calderini, D. F. (2015). Volume, Water Content, Epidermal Cell Area, and XTH5 Expression in Growing Grains of Wheat across Ploidy Levels. F. Crop Res. 173, 30–40. doi:10.1016/J.FCR.2014.12.010
Nakashima, K., and Yamaguchi-Shinozaki, K. (2013). ABA Signaling in Stress-Response and Seed Development. Plant Cell Rep. 327 (32), 959–970. doi:10.1007/S00299-013-1418-1
Nakayama, A., Park, S., Zheng-Jun, X., Nakajima, M., and Yamaguchi, I. (2002). Immunohistochemistry of Active Gibberellins and Gibberellin-Inducible α-Amylase in Developing Seeds of Morning Glory. Plant Physiol. 129, 1045–1053. doi:10.1104/PP.010921
Nayak, S. N., Zhu, H., Varghese, N., Datta, S., Choi, H. K., Horres, R., et al. (2010). Integration of Novel SSR and Gene-Based SNP Marker Loci in the Chickpea Genetic Map and Establishment of New Anchor Points with Medicago Truncatula Genome. Theor. Appl. Genet. 120, 1415–1441. doi:10.1007/s00122-010-1265-1
Nelson, S. K., Ariizumi, T., and Steber, C. M. (2017). Biology in the Dry Seed: Transcriptome Changes Associated with Dry Seed Dormancy and Dormancy Loss in the Arabidopsis GA-insensitive Sleepy1-2 Mutant. Front. Plant Sci. 8, 1–21. doi:10.3389/fpls.2017.02158
Ozga, J. A., Yu, J., and Reinecke, D. M. (2003). Pollination-, Development-, and Auxin-specific Regulation of Gibberellin 3β-Hydroxylase Gene Expression in Pea Fruit and Seeds. Plant Physiol. 131, 1137–1146. doi:10.1104/PP.102.015974
Parry, G., Calderon-Villalobos, L. I., Prigge, M., Peret, B., Dharmasiri, S., Itoh, H., et al. (2009). Complex Regulation of the TIR1/AFB Family of Auxin Receptors. Proc. Natl. Acad. Sci. 106, 22540–22545. doi:10.1073/PNAS.0911967106
Pattanayak, A., Roy, S., Sood, S., Iangrai, B., Banerjee, A., Gupta, S., et al. (2019). Rice Bean: A Lesser Known Pulse with Well-Recognized Potential. Planta 250, 873–890. doi:10.1007/s00425-019-03196-1
Pazhamala, L. T., Agarwal, G., Bajaj, P., Kumar, V., Kulshreshtha, A., Saxena, R. K., et al. (2016). Deciphering Transcriptional Programming during Pod and Seed Development Using RNA-Seq in Pigeonpea (Cajanus cajan). PLoS One 11, e0164959. doi:10.1371/JOURNAL.PONE.0164959
Peng, L., Qian, L., Wang, M., Liu, W., Song, X., Cheng, H., et al. (2021). Comparative Transcriptome Analysis during Seeds Development between Two Soybean Cultivars. PeerJ 9, 1–20. doi:10.7717/peerj.10772
Pradhan, S., Bandhiwal, N., Shah, N., Kant, C., Gaur, R., and Bhatia, S. (2014). Global Transcriptome Analysis of Developing Chickpea (Cicer arietinum L.) Seeds. Front. Plant Sci. 5, 1–14. doi:10.3389/fpls.2014.00698
Qi, Z., Zhang, Z., Wang, Z., Yu, J., Qin, H., Mao, X., et al. (2018). Meta-analysis and Transcriptome Profiling Reveal Hub Genes for Soybean Seed Storage Composition during Seed Development. Plant Cell Environ. 41, 2109–2127. doi:10.1111/pce.13175
Rai, M. K., Phulwaria, M., and Shekhawat, N. S. (2013). Transferability of Simple Sequence Repeat (SSR) Markers Developed in Guava (Psidium guajava L.) to Four Myrtaceae Species. Mol. Biol. Rep. 40, 5067–5071. doi:10.1007/s11033-013-2608-1
Raizada, A., and Jegadeesan, S. (2020). Comparative Transcriptomic Analysis Revealed Complex 2 Molecular Mechanisms Underlying Pests, Pathogens Resistance 3 and Seed Development in Wild and Cultivated Blackgram. bioRxiv
Rashotte, A. M., Poupart, J., Waddell, C. S., and Muday, G. K. (2003). Transport of the Two Natural Auxins, Indole-3-Butyric Acid and Indole-3-Acetic Acid, in Arabidopsis. Plant Physiol. 133, 761–772. doi:10.1104/PP.103.022582
Reinhardt, D., Mandel, T., and Kuhlemeier, C. (2000). Auxin Regulates the Initiation and Radial Position of Plant Lateral Organs. Plant Cell 12, 507–518. doi:10.1105/TPC.12.4.507
Riefler, M., Novak, O., Strnad, M., and Schmülling, T. (2006). Arabidopsis Cytokinin Receptor Mutants Reveal Functions in Shoot Growth, Leaf Senescence, Seed Size, Germination, Root Development, and Cytokinin Metabolism. Plant Cell 18, 40–54. doi:10.1105/TPC.105.037796
Robert, C., Noriega, A., Tocino, Á., and Cervantes, E. (2008). Morphological Analysis of Seed Shape in Arabidopsis thaliana Reveals Altered Polarity in Mutants of the Ethylene Signaling Pathway. J. Plant Physiol. 165, 911–919. doi:10.1016/J.JPLPH.2007.10.005
Ruuska, S. A., Schwender, J., and Ohlrogge, J. B. (2004). The Capacity of Green Oilseeds to Utilize Photosynthesis to Drive Biosynthetic Processes. Plant Physiol. 136, 2700–2709. doi:10.1104/PP.104.047977
Schmutz, J., McClean, P. E., Mamidi, S., Wu, G. A., Cannon, S. B., Grimwood, J., et al. (2014). A Reference Genome for Common Bean and Genome-wide Analysis of Dual Domestications. Nat. Genet. 467 46, 707–713. doi:10.1038/ng.3008
Schruff, M. C., Spielman, M., Tiwari, S., Adams, S., Fenby, N., and Scott, R. J. (2006). The Auxin Response Factor 2 Gene of Arabidopsis Links Auxin Signalling, Cell Division, and the Size of Seeds and Other Organs. Development 133, 251–261. doi:10.1242/DEV.02194
Shen, C., Bai, Y., Wang, S., Zhang, S., Wu, Y., Chen, M., et al. (2010). Expression Profile of PIN, AUX/LAX and PGP Auxin Transporter Gene Families in Sorghumbicolorunder Phytohormone and Abiotic Stress. FEBS J. 277, 2954–2969. doi:10.1111/J.1742-4658.2010.07706.X
Shlamovitz, N., Ziv, M., and Zamski, E. (19951995). Light, Dark and Growth Regulator Involvement in Groundnut (Arachis hypogaea L.) Pod Development. Plant Growth Regul. 16, 37–42. doi:10.1007/BF00040505
Singh, D. P., Jermakow, A. M., and Swain, S. M. (2002). Gibberellins Are Required for Seed Development and Pollen Tube Growth in Arabidopsis. Plant Cell 14, 3133–3147. doi:10.1105/TPC.003046
Sinha, P., Bajaj, P., Pazhamala, L. T., Nayak, S. N., Pandey, M. K., Chitikineni, A., et al. (2020). Arachis hypogaea Gene Expression Atlas for Fastigiata Subspecies of Cultivated Groundnut to Accelerate Functional and Translational Genomics Applications. Plant Biotechnol. J. 18, 2187–2200. doi:10.1111/PBI.13374
Souframanien, J., and Reddy, K. S. (2015). De Novo Assembly, Characterization of Immature Seed Transcriptome and Development of Genic-SSR Markers in Black Gram [Vigna mungo (L.) Hepper]. PLoS One 10, e0128748. doi:10.1371/JOURNAL.PONE.0128748
Suanum, W., Somta, P., Kongjaimun, A., Yimram, T., Kaga, A., Tomooka, N., et al. (2016). Co-Localization of QTLs for Pod Fiber Content and Pod Shattering in F 2 and Backcross Populations between Yardlong Bean and Wild Cowpea. Mol. Breed. 36, 1–11. doi:10.1007/S11032-016-0505-8
Sudheesh, S., Sawbridge, T. I., Cogan, N. O., Kennedy, P., Forster, J. W., and Kaur, S. (2015). De Novo Assembly and Characterisation of the Field Pea Transcriptome Using RNA-Seq. BMC Genomics 16, 611. doi:10.1186/S12864-015-1815-7
Takahashi, T., Gasch, A., Nishizawa, N., and Chua, N. H. (1995). The DIMINUTO Gene of Arabidopsis is Involved in Regulating Cell Elongation. Genes Dev. 9, 97–107. doi:10.1101/GAD.9.1.97
Takahashi, Y., Sakai, H., Yoshitsu, Y., Muto, C., Anai, T., Pandiyan, M., et al. (2019). Domesticating Vigna Stipulacea: A Potential Legume Crop with Broad Resistance to Biotic Stresses. Front. Plant Sci. 10, 1607. doi:10.3389/FPLS.2019.01607
Tamaki, S., Matsuo, S., Hann, L. W., Yokoi, S., and Shimamoto, K. (2007). Hd3a Protein is a Mobile Flowering Signal in Rice. Science 316, 1033–1036. doi:10.1126/science.1141753
Tang, H., Cuevas, H. E., Das, S., Sezen, U. U., Zhou, C., Guo, H., et al. (2013). Seed Shattering in a Wild Sorghum is Conferred by a Locus Unrelated to Domestication. Proc. Natl. Acad. Sci. 110, 15824–15829. doi:10.1073/PNAS.1305213110
Tarkowski, P., Tarkowská, D., Novák, O., Mihaljević, S., Magnus, V., Strnad, M., et al. (2006). Cytokinins in the Perianth, Carpels, and Developing Fruit of Helleborus niger L. J. Exp. Bot. 57, 2237–2247. doi:10.1093/JXB/ERJ190
Tian, J., Isemura, T., Kaga, A., Vaughan, D. A., Tomooka, N., and McIntyre, C. L. (2013). Genetic Diversity of the rice Bean (Vigna Umbellata) Genepool as Assessed by SSR Markers. Genome 56, 717–727. doi:10.1139/gen-2013-0118
Tian, X., Li, S., Liu, Y., and Liu, X. (2016a). Transcriptomic Profiling Reveals Metabolic and Regulatory Pathways in the Desiccation Tolerance of Mungbean (Vigna radiata [L.] R. Wilczek). Front. Plant Sci. 7, 1–12. doi:10.3389/fpls.2016.01921
Tian, Y., Zhang, M., Hu, X., Wang, L., Dai, J., Xu, Y., et al. (2016b). Over-Expression of CYP78A98, a Cytochrome P450 Gene from Jatropha curcas L., Increases Seed Size of Transgenic Tobacco. Electron. J. Biotechnol. 19, 15–22. doi:10.1016/j.ejbt.2015.11.001
Walton, L. J., Kurepin, L. V., Yeung, E. C., Shah, S., Emery, R. J. N., Reid, D. M., et al. (2012). Ethylene Involvement in Silique and Seed Development of Canola, Brassica napus L. Plant Physiol. Biochem. 58, 142–150. doi:10.1016/J.PLAPHY.2012.06.016
Wan, L., Li, B., Lei, Y., Yan, L., Ren, X., Chen, Y., et al. (2017). Mutant Transcriptome Sequencing Provides Insights into Pod Development in Peanut (Arachis hypogaea L.). Front. Plant Sci. 0, 1900. doi:10.3389/FPLS.2017.01900
Watcharatpong, P., Kaga, A., Chen, X., and Somta, P. (2020). Narrowing Down a Major QTL Region Conferring Pod Fiber Contents in Yardlong Bean (Vigna unguiculata), a Vegetable Cowpea. Genes 11, 363. doi:10.3390/GENES11040363
Werner, T., Holst, K., Pörs, Y., Guivarc’h, A., Mustroph, A., Chriqui, D., et al. (2008). Cytokinin Deficiency Causes Distinct Changes of Sink and Source Parameters in Tobacco Shoots and Roots. J. Exp. Bot. 59, 2659–2672. doi:10.1093/JXB/ERN134
Xiang, J., Tang, S., Zhi, H., Jia, G., Wang, H., and Diao, X. (2017). Loose Panicle1 Encoding a Novel WRKY Transcription Factor, Regulates Panicle Development, Stem Elongation, and Seed Size in Foxtail Millet [Setaria italica (L.) P. Beauv.]. PLoS One 12, e0178730. doi:10.1371/JOURNAL.PONE.0178730
Xu, W., and Huang, W. (20172017). Calcium-Dependent Protein Kinases in Phytohormone Signaling Pathways. Int. J. Mol. Sci. 18, 2436. doi:10.3390/IJMS18112436,
Ye, J., Fang, L., Zheng, H., Zhang, Y., Chen, J., Zhang, Z., et al. (2006). WEGO: A Web Tool for Plotting GO Annotations. Nucleic Acids Res. 34, W293–W297. doi:10.1093/NAR/GKL031
Yi, F., Gu, W., Chen, J., Song, N., Gao, X., Zhang, X., et al. (2019). High Temporal-Resolution Transcriptome Landscape of Early maize Seed Development. Plant Cell 31, 974–992. doi:10.1105/tpc.18.00961
Zhang, D. F., Zhang, N., Zhong, T., Wang, C., Xu, M. L., and Ye, J. R. (2016). Identification and Characterization of the GH3 Gene Family in Maize. J. Integr. Agric. 15, 249–261. doi:10.1016/S2095-3119(15)61076-0
Zhong, R., Kays, S. J., Schroeder, B. P., and Ye, Z.-H. (2002). Mutation of a Chitinase-Like Gene Causes Ectopic Deposition of Lignin, Aberrant Cell Shapes, and Overproduction of Ethylene. Plant Cell 14, 165–179. doi:10.1105/TPC.010278
Zhou, Y., Lu, D., Li, C., Luo, J., Zhu, B.-F., Zhu, J., et al. (2012). Genetic Control of Seed Shattering in Rice by the APETALA2 Transcription Factor Shattering Abortion1. Plant Cell 24, 1034–1048. doi:10.1105/TPC.111.094383
Zhu, W., Chen, X., Li, H., Zhu, F., Hong, Y., Varshney, R. K., et al. (2014). Comparative Transcriptome Analysis of Aerial and Subterranean Pods Development Provides Insights into Seed Abortion in Peanut. Plant Mol. Biol. 85, 395–409. doi:10.1007/s11103-014-0193-x
Zhu, Z., Chen, H., Xie, K., Liu, C., Li, L., Liu, L., et al. (2020). Characterization of Drought-Responsive Transcriptome during Seed Germination in Adzuki Bean (Vigna angularis L.) by PacBio SMRT and Illumina Sequencing. Front. Genet. 11, 1–14. doi:10.3389/fgene.2020.00996
Keywords: ricebean, seed size, hormone signaling, MapMan, SSR
Citation: Verma SK, Mittal S, Gayacharan , Wankhede DP, Parida SK, Chattopadhyay D, Prasad G, Mishra DC, Joshi DC, Singh M, Singh K and Singh AK (2022) Transcriptome Analysis Reveals Key Pathways and Candidate Genes Controlling Seed Development and Size in Ricebean (Vigna umbellata). Front. Genet. 12:791355. doi: 10.3389/fgene.2021.791355
Received: 08 October 2021; Accepted: 23 December 2021;
Published: 21 January 2022.
Edited by:
Aamir Raina, Aligarh Muslim University, IndiaReviewed by:
Mahesh Rao, Indian Council of Agricultural Research, IndiaSuresha Giriyapura Shivalingamurthy, Indian Council of Agricultural Research, Coimbatore, India
Khela Ram Soren, Indian Institute of Pulses Research (ICAR), India
Copyright © 2022 Verma, Mittal, Gayacharan, Wankhede, Parida, Chattopadhyay, Prasad, Mishra, Joshi, Singh, Singh and Singh. This is an open-access article distributed under the terms of the Creative Commons Attribution License (CC BY). The use, distribution or reproduction in other forums is permitted, provided the original author(s) and the copyright owner(s) are credited and that the original publication in this journal is cited, in accordance with accepted academic practice. No use, distribution or reproduction is permitted which does not comply with these terms.
*Correspondence: Amit Kumar Singh, amit.singh5@icar.gov.in
†Present address: Kuldeep Singh, International Crops Research Institute for the Semi-Arid Tropics, Hyderabad, India
‡These authors have contributed equally to this work and share first authorship